AI Bias: When Your Robot Overlord Has a Favorite Child
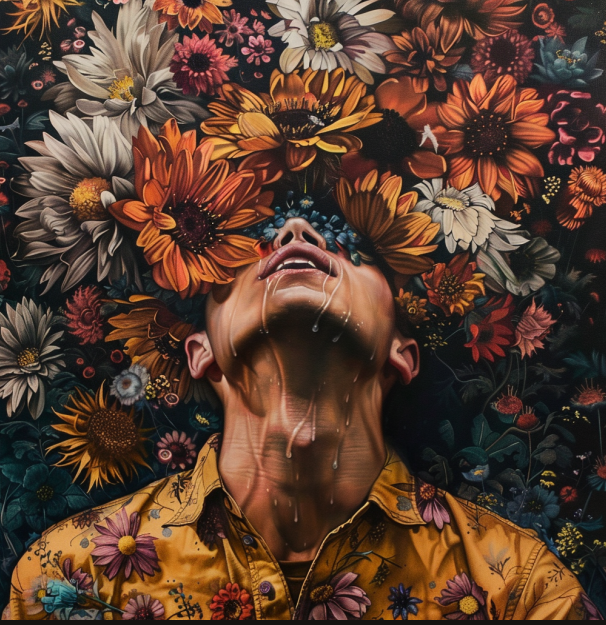
As artificial intelligence (AI) systems become increasingly prevalent in business decision-making, a critical issue has emerged: AI bias. This phenomenon occurs when AI systems produce results that unfairly privilege certain groups over others, often reflecting and amplifying existing societal prejudices. For business leaders, understanding and addressing AI bias is not just an ethical imperative but a crucial factor in ensuring the effectiveness and reliability of AI-driven processes.
The Hidden Prejudices of Algorithms
AI systems, despite their reputation for cold logic, are not immune to bias. In fact, they can exhibit prejudices that are as problematic as those of humans, if not more so due to their scale and reach. These biases typically stem from three main sources:
- Biased training data
- Flawed algorithm design
- Biased implementation or interpretation of results
Research indicates that up to 85% of AI projects show signs of bias or errors that could increase discriminatory outcomes. This statistic underscores the urgency of addressing bias in AI systems.
Real-World Impacts of AI Bias
The consequences of AI bias can be far-reaching and significant:
Hiring and Human Resources
AI-powered recruitment tools have shown biases against certain demographic groups, potentially perpetuating workplace inequalities. In one high-profile case, a major tech company had to scrap its AI recruiting tool after discovering it showed bias against female candidates.
Financial Services
Credit scoring algorithms have been found to disproportionately disadvantage minority groups, affecting their access to loans and financial products. This can have long-term economic impacts on individuals and communities.
Healthcare
AI systems used in healthcare have shown biases in diagnosing and recommending treatments for certain ethnic groups, potentially leading to disparities in health outcomes.
Strategies for Combating AI Bias
Addressing AI bias requires a multi-faceted approach:
Diverse Development Teams
Building AI systems with diverse teams can help identify and mitigate potential biases early in the development process. Companies with diverse AI teams report 33% fewer bias-related issues in their AI systems.
Rigorous Testing and Auditing
Regular audits of AI systems can help identify biases. This involves:
- Testing with diverse datasets
- Analyzing outcomes across different demographic groups
- Continuously monitoring system performance
Transparent AI
Promoting transparency in AI decision-making processes allows for better scrutiny and faster identification of biases. This includes:
- Documenting the data sources and methodologies used
- Providing explanations for AI-generated decisions
- Allowing for human oversight and intervention
Ethical AI Frameworks
Developing and adhering to ethical AI frameworks can guide the responsible development and deployment of AI systems. Key elements often include:
- Fairness and non-discrimination principles
- Accountability measures
- Privacy protections
The Business Case for Unbiased AI
Addressing AI bias is not just about ethical considerations; it makes good business sense:
- Enhanced Reputation: Companies known for fair and ethical AI practices can build trust with customers and stakeholders.
- Improved Decision-Making: Unbiased AI systems lead to more accurate and reliable insights, driving better business outcomes.
- Reduced Legal Risk: As regulations around AI fairness increase, addressing bias can help companies avoid potential legal issues.
- Expanded Market Reach: AI systems free from bias can better serve diverse customer bases, opening up new market opportunities.
Looking Ahead: The Future of Fair AI
As awareness of AI bias grows, we can expect to see:
- More sophisticated bias detection tools
- Increased regulation around AI fairness
- Growing demand for AI ethics experts in the business world
Companies that proactively address AI bias will be better positioned to harness the full potential of AI while maintaining ethical standards and public trust.
The challenge of AI bias reminds us that as we delegate more decisions to artificial intelligence, we must remain vigilant. Our robot overlords may not have favorites, but without careful oversight, they might just act like they do. By addressing bias head-on, businesses can ensure that their AI systems are not just powerful, but fair and trustworthy as well.