AI Ethics: A Roadmap for Responsible Innovation
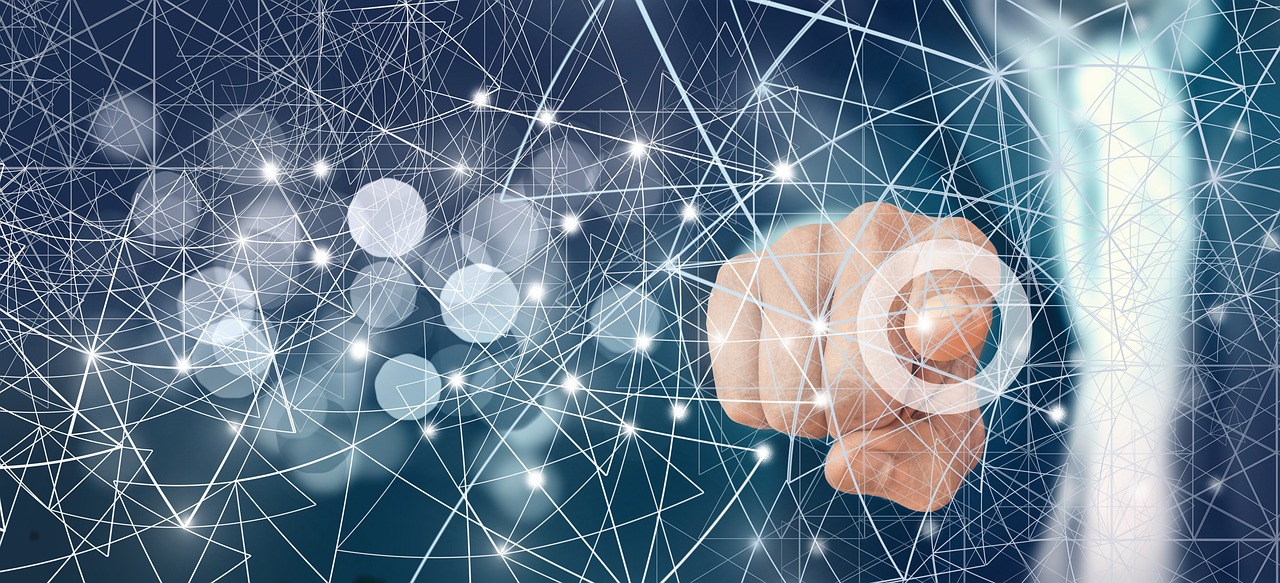
As artificial intelligence (AI) becomes increasingly integrated into our daily lives, it is transforming industries, reshaping economies, and influencing social norms. Yet, with its tremendous potential comes a responsibility to navigate complex ethical dilemmas. From concerns about privacy and bias to the broader impact on jobs and society, the ethical use of AI is a topic that cannot be overlooked. As a result, establishing a clear framework for responsible innovation is essential for building trust and ensuring that AI serves the collective good.
Understanding AI Ethics: More Than Compliance
AI ethics goes beyond regulatory compliance or corporate responsibility—it is about embedding ethical considerations into every stage of AI development and deployment. At its core, AI ethics involves assessing how AI impacts individuals and society, ensuring that its use aligns with fundamental human values such as fairness, transparency, and accountability. This means thinking not just about what AI can do, but what it should do.
The ethical challenges of AI arise from its unique characteristics. Unlike traditional software, AI systems can make decisions autonomously, learn from data, and adapt over time. This dynamic nature raises questions about how to ensure these systems behave responsibly, avoid unintended consequences, and do not perpetuate harmful biases.
AI ethics is not just a theoretical concern—it has real-world implications. For example, a recruitment algorithm that inadvertently favors certain demographics, a facial recognition system used in ways that infringe on privacy, or a predictive policing tool that reinforces historical biases all pose serious ethical risks. These scenarios highlight the need for ethical guidelines that go beyond technical specifications to consider broader social impacts.
Core Principles of AI Ethics
To create a framework for ethical AI, organizations must start by defining the core principles that will guide their approach. These principles serve as a foundation for decision-making, helping to ensure that AI technologies are developed and used in ways that align with societal values and expectations.
Fairness and Non-Discrimination
AI systems should treat all individuals fairly and without discrimination. This principle is particularly important in applications that affect people’s lives, such as hiring, lending, and law enforcement. Bias in AI can arise from various sources, including biased training data, flawed model assumptions, or skewed sampling. If left unchecked, these biases can lead to unfair outcomes and reinforce social inequalities.
Ensuring fairness requires a proactive approach to identifying and mitigating bias. This involves using diverse and representative datasets, regularly auditing algorithms for biased outcomes, and implementing mechanisms to allow affected individuals to contest decisions.
Transparency and Explainability
AI systems should be transparent, and their decision-making processes should be understandable to those affected by them. This principle, known as “explainability,” is essential for building trust in AI. When AI decisions have significant consequences—such as approving a loan or diagnosing a health condition—users should be able to understand why a particular decision was made.
However, achieving transparency in complex AI models, especially in deep learning systems that operate as “black boxes,” is a technical challenge. To address this, developers are exploring methods for making AI more interpretable, such as using simpler models, visualizing decision pathways, or employing post-hoc explanation techniques that shed light on how a decision was reached.
Accountability and Responsibility
Organizations that develop or deploy AI systems must be accountable for the outcomes of those systems. This means taking responsibility for the actions and decisions made by AI, whether positive or negative. Accountability is particularly crucial in cases where AI is used in sensitive contexts, such as healthcare, finance, or criminal justice.
Establishing accountability requires clarity about who is responsible for AI decisions and outcomes—the developers, the organizations that deploy the AI, or the users themselves. It also involves creating clear lines of responsibility and implementing mechanisms for addressing and correcting harmful outcomes.
Privacy and Data Protection
AI systems often rely on large volumes of personal data to function effectively. Ensuring that these systems respect individuals’ privacy and adhere to data protection standards is a critical ethical consideration. This involves implementing robust data governance practices, ensuring that data is collected and used with proper consent, and anonymizing data where possible to protect user identities.
Privacy is particularly challenging in AI due to the risk of re-identification, where seemingly anonymized data can be cross-referenced with other datasets to reveal personal information. To safeguard privacy, organizations must adopt a “privacy by design” approach, embedding data protection measures into the core of AI systems from the outset.
Safety and Reliability
AI systems must be designed to operate safely and reliably, even under unexpected conditions. This principle is especially important in applications like autonomous vehicles, healthcare, or industrial automation, where malfunctions could have serious consequences. Ensuring safety involves rigorous testing, continuous monitoring, and the ability to shut down or override AI systems in case of failures or unforeseen behaviors.
Reliability also extends to ensuring that AI systems perform consistently across different environments and use cases. This requires thorough validation and an ongoing commitment to maintaining and updating the system as new challenges emerge.
Human-Centric Design
AI should be designed to enhance human capabilities and well-being, not replace or undermine them. This principle advocates for a “human-in-the-loop” approach, where humans maintain meaningful control over AI systems, particularly in decision-making processes that affect people’s lives. It also emphasizes that AI should complement human skills and support users, rather than dictating actions or reducing human agency.
Human-centric design is especially important in areas like healthcare, where AI can assist clinicians but should not make critical decisions independently. Ensuring that AI remains a tool for human empowerment rather than a substitute for human judgment is key to maintaining ethical boundaries.
Building a Roadmap for Responsible Innovation
Creating an ethical AI framework involves more than just establishing guiding principles—it requires a structured approach to embedding these values into the design, development, and deployment of AI systems. Below are some strategies for building a roadmap for responsible innovation.
1. Establishing Ethical Guidelines and Policies
Organizations should start by developing clear ethical guidelines that outline their commitment to responsible AI. These guidelines should be informed by the core principles discussed above and tailored to the organization’s specific context and use cases. Ethical guidelines provide a foundation for consistent decision-making and set expectations for how AI technologies will be used.
Once established, these guidelines should be formalized into policies that are integrated into the organization’s workflows. This ensures that ethical considerations are not an afterthought but a central component of AI development from the beginning.
2. Creating Multi-Disciplinary Ethics Committees
AI ethics is inherently interdisciplinary, involving technical, legal, philosophical, and social dimensions. To ensure that diverse perspectives are considered, organizations should create multi-disciplinary ethics committees that include not only AI developers but also ethicists, legal experts, and representatives from affected communities.
These committees can provide guidance on complex ethical issues, review AI projects for compliance with ethical standards, and serve as a forum for discussing emerging challenges. Establishing such a body helps organizations navigate difficult ethical decisions and ensures that AI is developed in a way that respects diverse values and viewpoints.
3. Implementing Ethical Design Practices
Ethical design practices involve embedding ethical considerations directly into the technical design of AI systems. This can include developing algorithms that are transparent and interpretable, using fairness metrics to detect and mitigate bias, and building mechanisms for user feedback and contestability.
Ethical design should also consider the broader social impacts of AI. For example, when designing a predictive policing tool, it is important to account for the potential for racial bias and ensure that the tool does not disproportionately impact certain communities.
4. Providing Ongoing Training and Awareness
Creating an ethical AI culture requires more than just policies and guidelines—it requires training and awareness at every level of the organization. Regular workshops, seminars, and training sessions can help employees understand the ethical implications of AI and how to apply ethical principles in their work.
Training should cover not only technical aspects, such as detecting bias or ensuring transparency, but also broader topics like the societal impacts of AI and emerging ethical dilemmas. Building a shared understanding of ethical values ensures that employees at all levels can contribute to responsible AI development.
5. Engaging with External Stakeholders
AI ethics is not just an internal concern—it has broad implications for society. Engaging with external stakeholders, such as regulators, advocacy groups, and the public, helps organizations understand the societal context of their AI applications and align their practices with broader societal values.
Stakeholder engagement can take the form of public consultations, collaborations with academic researchers, or participation in industry-wide ethics initiatives. By engaging with diverse voices, organizations can build more inclusive and ethical AI systems that are aligned with the needs and concerns of the communities they serve.
The Future of AI Ethics
As AI continues to evolve, so too will the ethical challenges it presents. Building a roadmap for responsible innovation requires more than a one-time effort—it demands ongoing vigilance, adaptation, and dialogue. Organizations must be prepared to revisit their ethical frameworks as new use cases, technologies, and societal expectations emerge.
Ultimately, the goal of AI ethics is not to stifle innovation but to guide it in a direction that maximizes benefits while minimizing harm. By adopting a proactive approach to ethical AI, organizations can ensure that they are not just developing cutting-edge technology, but doing so in a way that upholds the values of fairness, transparency, and respect for human rights.
Setting the Standard for Ethical AI
The path to responsible AI is challenging, but it is also an opportunity for leadership. Organizations that prioritize ethical considerations in their AI development will be better positioned to build trust, navigate regulatory landscapes, and create technologies that serve the greater good. In doing so, they can set the standard for what responsible innovation looks like in an increasingly AI-driven world.