AI Ethics Auditing: Ensuring Algorithmic Accountability
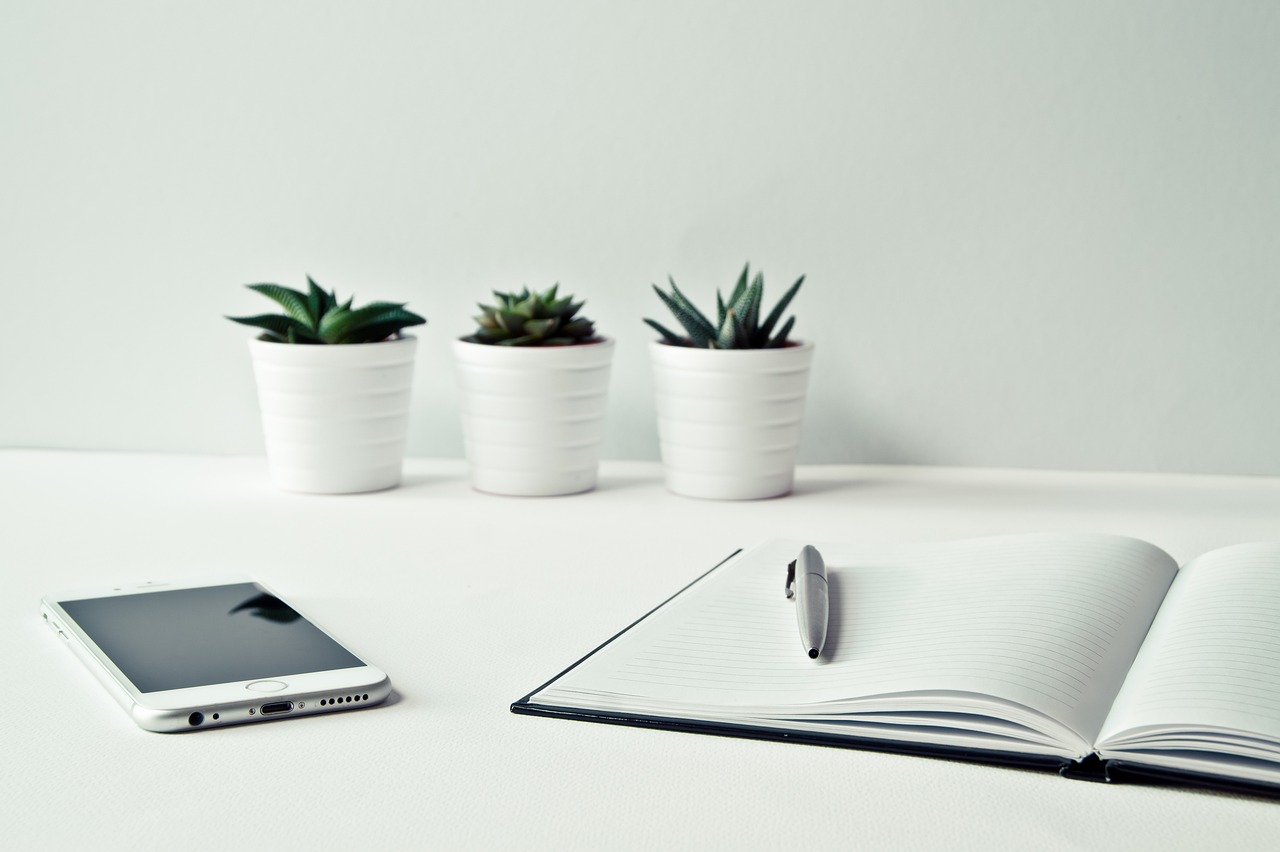
As artificial intelligence (AI) systems increasingly influence critical decisions in our lives, from loan approvals to hiring processes, the need for algorithmic accountability has never been more pressing. AI ethics auditing has emerged as a crucial tool in ensuring that AI systems are fair, transparent, and aligned with human values.
The Imperative for AI Ethics Auditing
AI technologies, while powerful, can perpetuate biases, make opaque decisions, or produce unintended consequences. Without proper oversight, these issues can lead to discrimination, privacy violations, and erosion of public trust.
Studies indicate that regular ethical audits of AI systems can significantly reduce the risk of harmful outcomes and improve overall system performance. As AI becomes more pervasive, the ability to conduct thorough ethics audits is becoming a critical competency for organizations.
Key Components of AI Ethics Auditing
1. Bias Detection and Mitigation
A fundamental aspect of AI ethics auditing is identifying and addressing biases in AI systems. This involves:
- Analyzing training data for underrepresentation or historical biases
- Testing AI outputs across different demographic groups
- Implementing techniques to mitigate detected biases
2. Transparency and Explainability Assessment
Ethical AI systems should be transparent in their decision-making processes. Audits should evaluate:
- The interpretability of AI models
- The clarity of explanations provided for AI decisions
- The extent to which the AI system’s workings can be understood by stakeholders
3. Privacy and Data Protection Evaluation
Audits must ensure that AI systems respect user privacy and comply with data protection regulations. This includes assessing:
- Data collection and storage practices
- Implementation of data minimization principles
- Robustness of data anonymization techniques
4. Fairness and Non-Discrimination Analysis
AI ethics audits should verify that AI systems produce fair outcomes. This involves:
- Testing for disparate impact across protected groups
- Evaluating the definition and implementation of fairness metrics
- Assessing the system’s performance in edge cases and minority groups
5. Safety and Reliability Checks
Ethical AI must be safe and reliable. Audits should examine:
- The robustness of AI systems against adversarial attacks
- The presence and effectiveness of fail-safe mechanisms
- The system’s performance under various stress scenarios
The Process of Conducting AI Ethics Audits
Effective AI ethics auditing follows a structured process:
1. Scope Definition
Clearly define the scope of the audit, including which systems, processes, and decisions will be examined.
2. Stakeholder Engagement
Identify and engage relevant stakeholders, including developers, users, and potentially affected communities.
3. Documentation Review
Examine all relevant documentation, including data sources, model architectures, and decision-making processes.
4. Technical Testing
Conduct thorough technical tests, including bias detection algorithms, fairness assessments, and security evaluations.
5. Impact Assessment
Evaluate the real-world impact of the AI system, considering both intended and unintended consequences.
6. Reporting and Recommendations
Produce a comprehensive report detailing findings and providing actionable recommendations for improvement.
7. Follow-up and Monitoring
Implement a plan for ongoing monitoring and periodic re-auditing to ensure continuous ethical compliance.
Challenges in AI Ethics Auditing
While crucial, implementing effective AI ethics audits comes with challenges:
- Complexity of AI Systems: The intricate nature of many AI systems can make thorough auditing difficult.
- Lack of Standardization: There’s currently no universally accepted standard for AI ethics audits.
- Resource Intensiveness: Comprehensive audits can be time-consuming and require specialized expertise.
- Evolving Ethical environment: As AI capabilities advance, new ethical considerations continually emerge.
The Business Case for AI Ethics Auditing
Despite these challenges, there’s a strong business case for investing in AI ethics auditing:
- Risk Mitigation: Identifying and addressing ethical issues early can prevent costly mistakes and legal issues.
- Enhanced Trust: Demonstrating commitment to ethical AI can boost user trust and brand reputation.
- Regulatory Compliance: As AI regulations change, regular audits can help ensure ongoing compliance.
- Improved Performance: Addressing biases and ethical issues often leads to better overall AI performance.
The Future of AI Ethics Auditing
As the field of AI ethics matures, we can expect several developments:
- Standardized Frameworks: The emergence of widely accepted standards and methodologies for AI ethics audits.
- Automated Auditing Tools: Development of AI-powered tools to assist in the auditing process.
- Regulatory Requirements: Increasing regulatory pressure mandating regular AI ethics audits.
- Third-Party Certification: The rise of independent bodies offering AI ethics certifications.
AI ethics auditing is not just a technical exercise—it’s a crucial step in ensuring that AI systems align with human values and societal expectations. As AI continues to shape our world, the ability to conduct thorough, effective ethics audits will be key to harnessing the benefits of AI while mitigating its risks.
By embracing AI ethics auditing, organizations can not only ensure compliance and mitigate risks but also lead the way in developing AI systems that are trustworthy, fair, and beneficial to society. In the AI era, ethical considerations are not a constraint on innovation, but a catalyst for creating AI systems that truly serve humanity’s best interests.