AI Governance Models: Charting the Course for Machine Morality
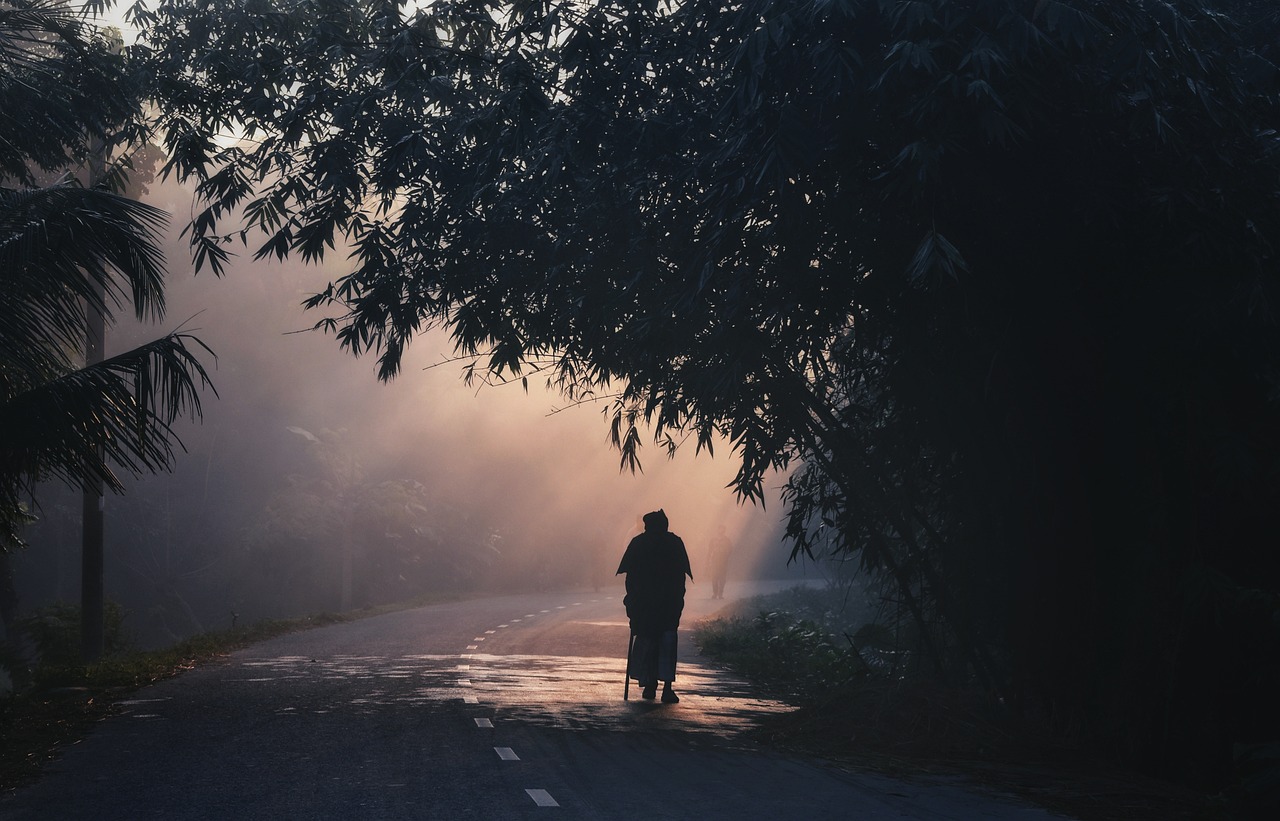
What Are AI Governance Models?
AI governance models are frameworks and policies that oversee the design, deployment, and management of artificial intelligence. They ensure that AI systems operate ethically, transparently, and responsibly, balancing technological advancement with societal values. These models are essential for establishing accountability, managing risks, and preventing misuse.
AI governance touches on many areas—legal frameworks, technical standards, and ethical guidelines. At its heart lies the challenge of integrating human morality into automated systems. Machine morality refers to the ability of AI to make decisions aligned with ethical principles, often under complex circumstances that require trade-offs. Designing effective governance models is crucial for ensuring AI remains a tool for good, serving the interests of humanity.
Why AI Governance Is Critical
AI technologies are increasingly embedded in essential areas of life, including healthcare, finance, education, and law enforcement. Without proper governance, AI can perpetuate biases, invade privacy, and make unfair or harmful decisions. Governance frameworks ensure that AI systems are accountable to the public, remain aligned with human values, and foster trust among users.
As AI becomes more autonomous, the need for governance becomes urgent. Complex decisions—such as which treatment to recommend for a patient or how an autonomous vehicle responds to a potential accident—require oversight to ensure fairness, transparency, and safety. Machine morality aims to embed ethical principles in these systems to guide them through such dilemmas responsibly.
Key Components of AI Governance Models
AI governance encompasses a broad spectrum of principles, regulations, and processes. Below are the essential elements that define robust governance models.
1. Transparency and Explainability
Transparency ensures that AI systems are understandable to stakeholders, including users, developers, and regulators. Explainability refers to the ability to interpret and communicate how an AI system arrives at its decisions.
Opaque algorithms can erode trust, especially in high-stakes sectors like healthcare or finance. Governance frameworks require companies to document their algorithms and make them auditable. Public institutions adopting AI should also be transparent about its use and decision-making logic to maintain accountability.
2. Fairness and Bias Mitigation
AI systems often reflect the biases present in the data they are trained on. Governance models must include processes to identify, measure, and mitigate bias to prevent discrimination. Fairness ensures that AI decisions do not disproportionately harm specific groups based on race, gender, socioeconomic status, or other attributes.
Regular audits and diverse training datasets help minimize bias, but fairness is a moving target. Governance frameworks must continuously evolve to address new forms of discrimination and ensure inclusivity across AI applications.
3. Accountability and Liability
Governance frameworks define who is responsible when AI systems malfunction or produce harmful outcomes. Clear accountability is essential in cases involving financial loss, discrimination, or harm caused by autonomous systems. These models establish rules for assigning liability among developers, operators, and users.
This component is particularly important in high-risk AI applications, such as self-driving cars or automated legal systems, where unintended consequences can have serious implications.
4. Privacy and Data Protection
AI systems rely heavily on large datasets, often containing sensitive personal information. Governance models must ensure that data collection, storage, and use adhere to privacy regulations. The use of data must align with principles such as consent, minimal data usage, and anonymization to safeguard individual rights.
Frameworks like the General Data Protection Regulation (GDPR) in the European Union set global standards for privacy, requiring transparency in data handling and empowering users with control over their data.
5. Safety and Security
AI systems, particularly autonomous ones, must operate safely under varying conditions. Governance frameworks establish safety standards for the development and testing of AI systems to minimize risks. Security protocols are also essential to protect AI systems from cyberattacks, data breaches, and unauthorized tampering.
Governments and organizations often require AI systems to undergo extensive testing and certification to ensure they are safe before deployment. In critical applications, such as defense or healthcare, these standards are even more rigorous.
Models of AI Governance in Practice
Governments, private companies, and international organizations are experimenting with different governance models to regulate AI effectively. Below are some prominent models shaping the global conversation around AI governance.
1. Regulatory Frameworks
Several countries have introduced AI-specific regulations to address ethical and legal concerns. The European Union’s AI Act aims to classify AI systems based on their risk level, with stricter requirements for high-risk applications such as biometric surveillance and autonomous vehicles.
In the United States, governance efforts have focused more on sector-specific regulation. For example, the Algorithmic Accountability Act requires companies to conduct impact assessments of their automated systems. China, on the other hand, emphasizes strict government control over AI, balancing innovation with national security priorities.
2. Industry Self-Regulation
In the absence of comprehensive government regulations, many companies have adopted voluntary governance frameworks. Google’s AI Principles and Microsoft’s Responsible AI Standards outline internal guidelines for the ethical use of AI. These initiatives focus on transparency, fairness, and accountability, though they remain limited by the lack of external oversight.
While self-regulation demonstrates proactive leadership, critics argue that external governance bodies are necessary to ensure compliance and prevent conflicts of interest.
3. International Governance Efforts
AI governance is inherently global, requiring coordination across countries to address cross-border challenges. The OECD AI Principles provide a framework for promoting innovation while safeguarding human rights and democratic values. The United Nations has also launched AI governance initiatives, focusing on using AI for sustainable development and mitigating the risks of autonomous weapons.
International cooperation ensures that AI governance frameworks align with global norms, preventing regulatory fragmentation that could hinder innovation or create loopholes.
The Challenge of Machine Morality
Embedding morality into AI systems presents a significant challenge. AI models cannot understand ethics the way humans do, making it difficult to teach them how to handle nuanced moral dilemmas. For example, a self-driving car may need to decide between protecting the passenger or avoiding harm to a pedestrian. These “trolley problems” illustrate the complexity of designing ethical algorithms.
Machine morality requires interdisciplinary collaboration, involving ethicists, engineers, and social scientists. Governance models must define clear ethical principles, but these principles can vary across cultures and contexts, complicating the task further. Some initiatives propose the use of value alignment techniques, which aim to align AI behavior with human values through carefully curated training data and feedback loops.
Collaboration as the Key to Effective AI Governance
Effective AI governance requires collaboration among governments, private companies, researchers, and civil society organizations. Governments must create enforceable regulations, while the private sector provides technical expertise. Academic institutions and advocacy groups can offer ethical insights, ensuring governance frameworks reflect societal values.
Public participation is also essential. Engaging diverse communities in conversations about AI governance ensures that marginalized voices are heard and potential harms are addressed proactively. Governance efforts must be inclusive, transparent, and adaptive to keep pace with the rapid evolution of AI technologies.
Building Trust Through Responsible Governance
Trust is a cornerstone of successful AI adoption. Governance models that prioritize transparency, fairness, and accountability foster public trust in AI systems. Without trust, individuals and organizations may resist using AI tools, limiting their potential for positive impact.
Building trust requires more than just compliance with rules—it demands a commitment to continuous learning and improvement. Governance models should include mechanisms for feedback, allowing stakeholders to report issues and suggest improvements. Regular reviews and updates ensure that governance frameworks remain relevant in a fast-changing technological landscape.
Charting the Future of AI Governance and Machine Morality
As AI continues to shape the future, governance models will play a critical role in guiding its development toward socially beneficial outcomes. Effective governance ensures that AI aligns with human values, operates transparently, and remains accountable to society. Achieving machine morality is a complex task, but it is essential to build AI systems that earn public trust and serve the common good.
The course of AI governance will require ongoing collaboration and thoughtful regulation. By balancing innovation with responsibility, society can harness the power of AI while minimizing its risks. Governance is not just a safeguard—it is the foundation for building AI systems that reflect the best of human morality and create a future where technology benefits everyone.