AI Pilot Projects: How to Avoid a Tech Car Crash
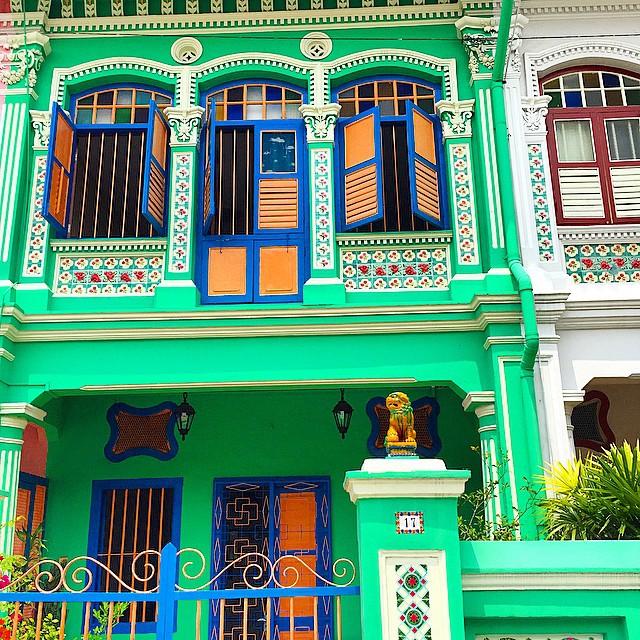
Artificial Intelligence (AI) has rapidly transformed from a futuristic concept to a tangible force driving innovation across industries. But with this excitement comes a stark reality: while AI has the potential to deliver game-changing outcomes, mismanaged AI pilot projects can end up causing a “tech car crash,” leading to significant financial losses and damaged credibility. Successfully navigating AI pilot projects requires not only technical expertise but also careful strategic planning. Understanding the pitfalls and how to avoid them can mean the difference between groundbreaking success and costly failure.
In this article, we will explore key strategies to ensure that your AI pilot projects run smoothly. From selecting the right pilot use case to aligning with business goals, we will break down the common mistakes companies make and how to steer clear of them.
The Importance of AI Pilot Projects
AI pilot projects serve as crucial stepping stones in evaluating how AI can be integrated into larger business processes. Before full-scale AI adoption, organizations need to test their AI models on a smaller scale. However, these pilot projects should not be treated as “just experiments” but as real-life business opportunities. The success of these pilots is often a predictor of how well AI will perform once scaled.
An AI pilot project gives organizations the chance to:
- Test the viability of AI solutions in real-world scenarios
- Identify technical and operational challenges
- Fine-tune algorithms and models
- Measure tangible business outcomes
Thus, an AI pilot project is the foundation on which broader AI success is built. Poorly executed pilots often lead to the wrong conclusions about AI’s value, resulting in wasted resources and missed opportunities. This is why understanding the framework of AI pilots is so important.
Choosing the Right AI Pilot Project Use Case
Choosing the wrong use case is one of the most common reasons AI pilot projects fail. When identifying a use case, many organizations jump to exciting but overly ambitious or highly complex AI applications. This often results in technical difficulties, lack of measurable outcomes, or outright project failure.
For a successful pilot, choose a use case that meets the following criteria:
- Clear Business Impact: The project should align directly with a key business goal, such as reducing operational costs or improving customer experience.
- Feasibility: The problem must be solvable using current AI technology, and your team must have access to the necessary data.
- Scalability: Ensure that if the pilot is successful, it can be easily scaled up without drastic changes to the model or infrastructure.
- Measurable Outcomes: The pilot must have clear metrics to assess performance, such as time saved, revenue increased, or error rates reduced.
Start small but meaningful. By choosing a manageable yet impactful use case, organizations can avoid stretching resources too thin and create a success story that will help justify further AI investment.
Aligning AI with Business Objectives
Too often, AI projects fail because they are treated as technical experiments rather than initiatives directly tied to business outcomes. Business leaders might not fully understand the potential or limitations of AI, while data science teams may lack insight into what the business actually needs. Bridging this gap is critical to avoiding a “tech car crash.”
Ensure that business leaders are involved in the pilot project from the start. They should provide input into what problems the AI is trying to solve, the value it can bring, and how success will be measured. Moreover, AI should not operate in isolation; it must complement and enhance existing workflows rather than disrupt them. This integration will make the pilot more relevant and easier to scale.
Business alignment requires:
- Close Collaboration: Teams across data science, IT, and business functions need to work together.
- Clear Communication: Set expectations on what the AI can achieve and in what timeframe.
- Focus on Value: Constantly keep the focus on how the AI pilot will generate value for the organization.
Data Readiness: The Fuel Behind AI
Data is the fuel that drives AI, but many companies enter into pilot projects with incomplete, poor-quality, or biased data. This can cause AI models to fail or underperform, resulting in a skewed view of AI’s effectiveness. To avoid this, organizations must ensure their data is clean, well-structured, and relevant to the pilot.
Considerations for data readiness include:
- Data Quality: Ensure your data is accurate, complete, and up-to-date.
- Data Volume: AI models, especially machine learning, require large datasets to function properly. Insufficient data can lead to unreliable models.
- Data Bias: AI models learn from historical data, so if your data contains biases, these biases will be reflected in the AI’s decisions. Ensure diversity and representation in your datasets to avoid ethical pitfalls.
Without proper data governance, an AI pilot is like driving a car without fuel—it’s bound to fail.
Managing Expectations: Avoiding the Hype Trap
The media often portrays AI as a magical solution to all business problems, leading to inflated expectations from stakeholders. This hype can be dangerous if not managed properly. Many AI pilot projects crash when they fail to deliver the “breakthroughs” promised by overenthusiastic leaders or misinformed teams.
AI is a powerful tool, but it is not a silver bullet. It has limitations, especially in a pilot phase. Set realistic expectations by clearly outlining the scope and potential outcomes of the pilot. Avoid overpromising and instead focus on incremental improvements and long-term gains.
By managing expectations, you can ensure that stakeholders remain invested in the project even if the initial results are less than spectacular.
Building a Cross-Functional AI Team
AI pilot projects require a multidisciplinary approach. It’s not enough to rely solely on data scientists or technical experts. Success hinges on collaboration between various departments, including business leaders, IT specialists, data engineers, and even marketing and legal teams. Each brings a unique perspective that ensures the AI pilot is aligned with the organization’s broader goals and can be operationalized effectively.
Key roles for your AI team include:
- Data Scientists: Develop the algorithms and machine learning models.
- Data Engineers: Ensure data pipelines are properly constructed and maintained.
- Business Analysts: Translate business objectives into AI requirements and vice versa.
- IT Support: Help with the deployment, integration, and scaling of the AI systems.
- Project Managers: Oversee the AI pilot and ensure it stays on track and within budget.
Without cross-functional input, an AI pilot can easily get derailed by technical challenges or fail to meet business needs.
Setting KPIs for AI Success
To assess whether your AI pilot project is on track, it is essential to establish clear Key Performance Indicators (KPIs). These KPIs should be aligned with both the technical performance of the AI system and the business outcomes it aims to achieve. Common AI-related KPIs might include:
- Accuracy of Predictions: How often the AI model is correct in its decisions.
- Reduction in Operational Costs: A measurable decrease in costs due to the AI solution.
- Time Savings: How much time has been saved by automating tasks previously handled by humans.
- Customer Satisfaction: Improvements in customer feedback or net promoter scores due to AI-driven improvements.
- Revenue Impact: Any noticeable increase in revenue tied to the AI’s contributions.
Tracking these KPIs ensures you have tangible metrics to assess success and justify further investment in AI.
Avoiding AI Talent Gaps
One of the hidden challenges of AI pilot projects is the talent gap. Many organizations underestimate the specialized skill sets required to manage and implement AI solutions. This can lead to poor project execution and ultimately failure. Hiring the right people is crucial to avoiding these pitfalls.
Given the competitive market for AI talent, companies should also consider upskilling existing employees or partnering with third-party vendors who can provide AI expertise. This approach ensures that you don’t overburden your internal team while still maintaining the technical proficiency needed for a successful pilot.
Monitoring and Iterating AI Models
AI is not a set-it-and-forget-it technology. Models need constant monitoring, tweaking, and retraining to ensure they continue to perform well as conditions change. For example, if your AI pilot uses predictive analytics to forecast demand, changes in the market or supply chain could cause the model to become outdated.
Establish processes for continuous monitoring and iteration. This might include:
- Regular Model Audits: Ensure the AI is still performing within acceptable parameters.
- Data Updates: Continuously feed fresh data into the model to keep it relevant.
- Feedback Loops: Use feedback from business users to refine the AI’s output.
Regular updates prevent your AI pilot from becoming obsolete or providing inaccurate results.