AI Project Pitfalls: The Implementation Maze
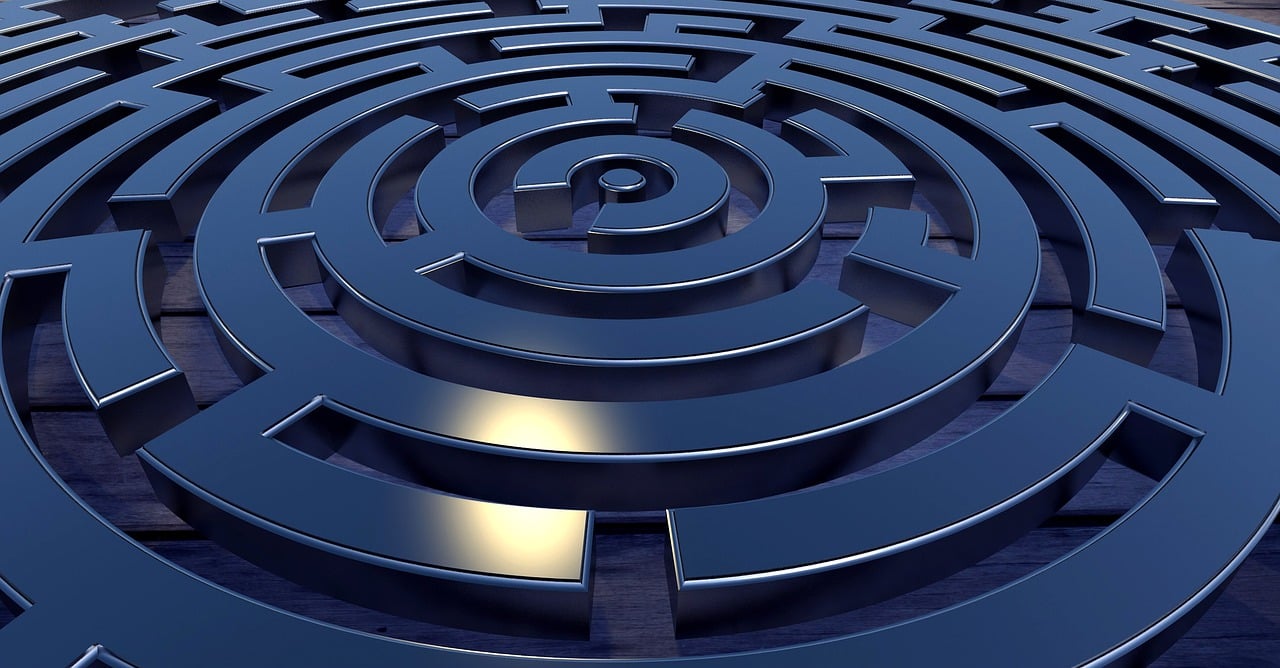
Artificial Intelligence (AI) has become a cornerstone of innovation across various industries. From automating business processes to enhancing customer experiences with predictive analytics, AI promises efficiency, productivity, and intelligence at an unprecedented scale. However, while the potential of AI is limitless, many organizations find themselves lost in the implementation maze. The journey from conceptualizing an AI project to realizing its tangible benefits is fraught with challenges, often leading to project delays, unfulfilled goals, or outright failures. This article explores the common pitfalls in AI projects and provides actionable insights on how to navigate the complex landscape of AI implementation.
The Excitement Around AI Projects
AI projects are often launched with immense excitement and anticipation. Organizations are eager to harness the power of machine learning, natural language processing, and computer vision to automate tasks, gain deeper insights from data, and create innovative products. Yet, despite this enthusiasm, the reality is stark—many AI projects fail to deliver on their promises. Studies show that up to 85% of AI projects fail to make it from the development stage into full-scale production.
So, why do so many AI projects struggle to succeed? The answer lies in the numerous technical, strategic, and organizational challenges that arise during the implementation phase. Let’s take a closer look at these obstacles.
Common AI Project Pitfalls
Implementing AI is not just about selecting the right algorithms or technology stack; it requires a deep understanding of the business context, data readiness, and the integration of AI into existing processes. Below are some of the most common pitfalls that organizations encounter when implementing AI projects.
1. Lack of Clear Business Objectives
One of the most significant reasons AI projects fail is the absence of well-defined business objectives. Too often, companies dive into AI initiatives without a clear understanding of the problem they are trying to solve. This lack of focus leads to a situation where AI is implemented for the sake of novelty, rather than to address a specific business challenge.
How to Avoid This Pitfall:
Start by defining the business goals of your AI project. Ask yourself questions like: What problem are we trying to solve? and How will AI add value to our current operations? Ensure that your AI project aligns with your overall business strategy. Having measurable objectives and key performance indicators (KPIs) in place will guide the project toward success.
2. Poor Data Quality and Accessibility
Data is the lifeblood of AI. However, many organizations underestimate the complexity of managing and preparing data for AI projects. AI systems are only as good as the data they are trained on, and poor data quality can lead to skewed or inaccurate results. Additionally, many organizations struggle with siloed data, where valuable information is scattered across multiple departments or systems, making it difficult to access and integrate.
How to Avoid This Pitfall:
Invest in data governance and data preparation early in the project. Ensure that data is clean, relevant, and representative of the problem you’re trying to solve. Break down data silos by fostering collaboration between departments and centralizing data storage. Implement processes for ongoing data cleaning and monitoring to ensure data remains accurate over time.
3. Underestimating the Complexity of AI Models
AI models, particularly those involving deep learning and advanced machine learning techniques, can be incredibly complex. Many organizations underestimate the expertise required to develop, train, and fine-tune these models. This often leads to projects becoming stalled in the development phase, as teams struggle to manage the complexity of the technology.
How to Avoid This Pitfall:
Ensure you have the right technical talent in place before starting an AI project. Hiring or partnering with experienced data scientists, machine learning engineers, and AI specialists is essential for navigating the complexity of AI model development. Additionally, consider starting with simpler models or off-the-shelf solutions before advancing to more complex AI systems.
4. Overlooking the Need for Continuous Monitoring and Maintenance
AI models are not static entities; they need to be continuously monitored, maintained, and updated. Many organizations make the mistake of thinking that once an AI model is deployed, the work is done. However, AI models can degrade over time as new data becomes available or as the environment in which the model operates changes. Without regular monitoring, AI systems can produce inaccurate or outdated results.
How to Avoid This Pitfall:
Establish a post-deployment plan for monitoring and maintaining AI models. This includes setting up systems to track the performance of AI models in real-time, as well as processes for retraining and updating models as needed. Regular audits of AI systems should be conducted to ensure that they are functioning as expected and delivering value.
5. Failure to Integrate AI with Existing Systems
Another major pitfall in AI projects is the failure to properly integrate AI models with existing systems and workflows. AI systems often need to interact with other software, databases, and operational processes to deliver value. However, organizations frequently underestimate the technical challenges involved in integration, leading to delays and additional costs.
How to Avoid This Pitfall:
Plan for integration from the outset of the project. Involve IT teams early in the process to assess how the AI system will interact with existing infrastructure. Choose AI tools and platforms that are compatible with your organization’s technology stack, and be prepared to invest in custom integrations if needed.
6. Insufficient Talent and Skills
AI is a rapidly evolving field, and the demand for skilled AI professionals far outstrips supply. Many organizations embark on AI projects without having the necessary in-house expertise, leading to stalled projects or suboptimal results. Hiring the right talent—data scientists, machine learning engineers, AI architects—can be a significant challenge for companies new to AI.
How to Avoid This Pitfall:
If your organization lacks AI expertise, consider partnering with external consultants or AI vendors who specialize in implementing AI solutions. Additionally, invest in upskilling your existing workforce through training programs and workshops. Creating a culture of continuous learning within your organization will help build long-term AI competency.
7. Ignoring Ethical and Regulatory Considerations
AI systems often make decisions that affect real people, whether in hiring processes, credit scoring, or healthcare diagnoses. Without careful consideration of the ethical implications of these decisions, AI projects can lead to biased outcomes, privacy violations, and regulatory issues. Furthermore, governments around the world are increasingly enacting regulations to govern the use of AI, and failure to comply with these regulations can result in fines and reputational damage.
How to Avoid This Pitfall:
Incorporate ethics and regulatory compliance into your AI project from the very beginning. Ensure that AI models are transparent, explainable, and free from bias. Implement robust data privacy and security practices to protect sensitive information. Stay informed about evolving regulations in your industry and region to ensure that your AI projects remain compliant.
Navigating the Implementation Maze
Successfully navigating the AI implementation maze requires a structured approach that addresses both technical and organizational challenges. Here are some key steps that can help you overcome AI project pitfalls:
Step 1: Start Small, Think Big
Rather than embarking on large, multi-faceted AI projects from the start, it’s often better to begin with a small, well-defined use case. A pilot project allows you to test AI solutions on a smaller scale, learn from the process, and make necessary adjustments before scaling up. Once you’ve demonstrated success in a pilot project, you can expand AI’s use across other areas of the organization.
Step 2: Foster Cross-Department Collaboration
AI projects often require input and data from multiple departments, including IT, marketing, operations, and finance. Fostering collaboration between departments is essential for ensuring that AI systems are designed and deployed in a way that aligns with broader business goals. Establish clear communication channels and create cross-functional teams to oversee AI initiatives.
Step 3: Prioritize User Adoption
Even the most sophisticated AI systems are only valuable if they are adopted by end-users. Many AI projects fail because employees are resistant to change or do not fully understand how to use the new technology. To overcome this barrier, invest in training and user education. Help employees understand how AI will benefit them in their day-to-day tasks and make the transition as smooth as possible.
Step 4: Focus on ROI
To ensure the long-term success of your AI project, always keep an eye on return on investment (ROI). AI projects should be focused on generating measurable business outcomes, whether that’s reducing costs, improving operational efficiency, or driving revenue growth. Regularly assess the financial impact of AI initiatives to ensure they are delivering value.
Conquering AI Project Challenges
While AI presents enormous opportunities for innovation, it also comes with its own set of challenges. By recognizing and addressing common pitfalls such as unclear objectives, poor data quality, lack of talent, and inadequate integration, organizations can avoid many of the common reasons AI projects fail. Successfully navigating the AI implementation maze requires careful planning, cross-functional collaboration, and a commitment to ongoing monitoring and improvement. By taking a strategic approach, organizations can unlock the full potential of AI and transform their operations in meaningful ways.