Building Public Trust in AI
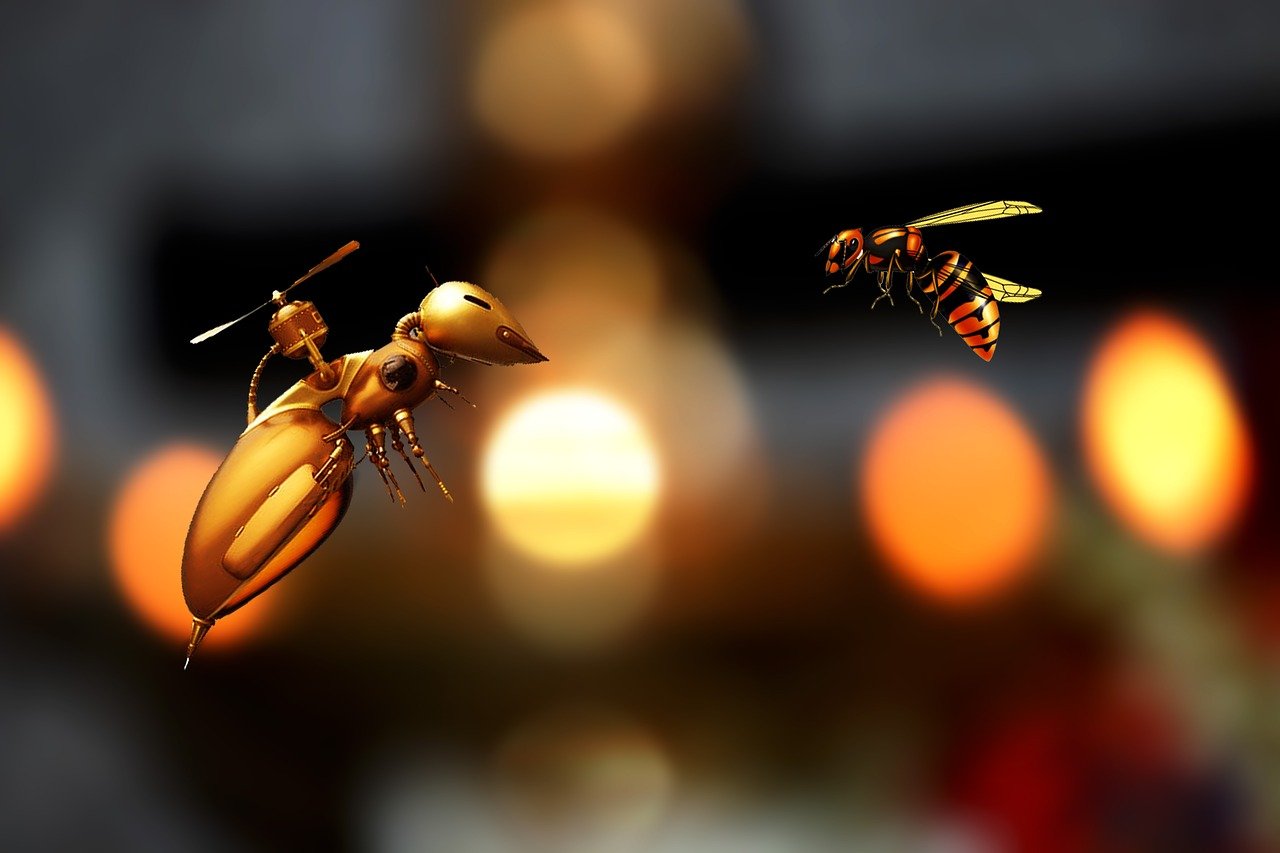
As artificial intelligence (AI) continues to reshape industries and society, a crucial challenge emerges: how to build and maintain public trust in these powerful technologies. Without trust, the full potential of AI may remain unrealized. This article explores strategies for fostering public confidence in AI systems and their applications.
The Trust Gap in AI
Despite rapid advancements in AI capabilities, public trust in these technologies remains fragile. Concerns include:
- Fear of job displacement due to automation
- Worries about privacy and data misuse
- Lack of understanding of AI decision-making processes
- Concerns about bias and fairness in AI systems
Addressing these concerns is crucial for the widespread acceptance and ethical deployment of AI technologies.
Strategies for Building Trust
Transparency in AI Development and Deployment
Openness about AI capabilities, limitations, and use cases is crucial for building trust.
Key actions:
- Clear communication about where and how AI is being used
- Disclosure of data sources and model training processes
- Regular updates on AI system performance and improvements
Explainable AI (XAI) Initiatives
Making AI decision-making processes more understandable to non-experts.
Approaches include:
- Developing interpretable AI models
- Creating user-friendly explanations of AI outputs
- Providing options for human review of AI decisions
Ethical AI Frameworks and Governance
Establishing clear guidelines and oversight mechanisms for AI development.
Components:
- Creation of AI ethics boards within organizations
- Adoption of industry-wide ethical AI standards
- Regular ethical audits of AI systems
Public Education and Engagement
Increasing AI literacy among the general public.
Initiatives:
- AI education programs in schools and communities
- Public demonstrations of AI capabilities and limitations
- Open forums for dialogue between AI developers and the public
Addressing Bias and Fairness
Actively working to identify and mitigate biases in AI systems.
Steps include:
- Diverse and representative data collection practices
- Regular bias audits of AI models
- Collaboration with affected communities in AI development
Responsible Data Handling
Prioritizing data privacy and security in AI applications.
Practices:
- Implementation of robust data protection measures
- Clear and accessible data usage policies
- Options for user control over personal data in AI systems
Human-AI Collaboration
Emphasizing AI as a tool to augment human capabilities rather than replace them.
Approaches:
- Designing AI systems with human oversight and input
- Showcasing successful human-AI partnerships
- Retraining programs for workers in AI-impacted industries
Case Studies in Building AI Trust
Healthcare AI
A major hospital system implemented an AI-assisted diagnosis tool, prioritizing transparency and doctor oversight. They provided clear explanations of the AI’s role to patients and allowed doctors to review and override AI recommendations. This approach led to improved diagnosis rates and high patient satisfaction.
Financial Services
A leading bank introduced an AI-powered credit scoring system. They offered customers insights into the factors influencing their credit scores and provided options to appeal decisions. This transparency led to increased customer trust and adoption of the AI-driven service.
The Business Case for Trustworthy AI
Building public trust in AI is not just an ethical imperative—it’s a business necessity. Benefits include:
- Increased adoption and user engagement with AI-powered products
- Enhanced brand reputation and customer loyalty
- Reduced regulatory risks and potential legal challenges
- Competitive advantage in an increasingly AI-driven market
Looking Ahead: The Future of AI Trust
As AI continues to grow, building and maintaining public trust will remain a critical challenge. Future developments may include:
- AI trust certifications and standards
- More sophisticated tools for AI explainability
- Increased integration of ethical considerations in AI education
- Evolution of regulations to address emerging AI trust issues
Building public trust in AI is an ongoing process that requires collaboration among technologists, policymakers, business leaders, and the public. By prioritizing transparency, ethics, and user empowerment, we can create an environment where AI technologies are not just powerful, but also trusted and embraced by society.
As we move forward, the goal is clear: to utilize the potential of AI in a way that aligns with human values, addresses societal needs, and earns the confidence of the public. Only then can we fully realize the benefits of AI advancements while mitigating its risks.