Building Trust through Explainable AI
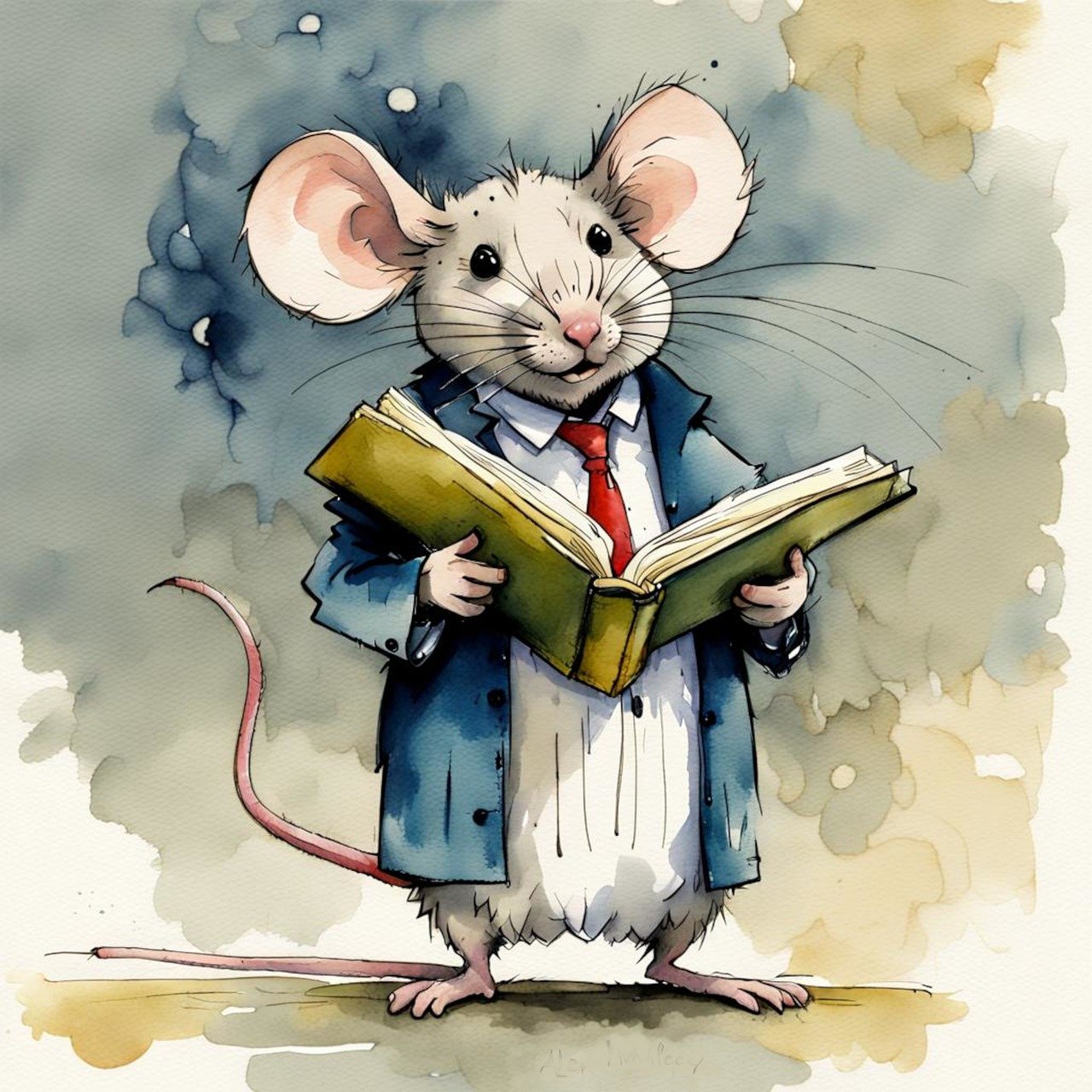
Artificial intelligence (AI) is transforming industries across the globe, from healthcare and finance to transportation and retail. However, as AI systems become more embedded in decision-making processes, concerns over their transparency, fairness, and accountability have grown. A key challenge is the “black box” nature of many AI models, where the logic behind their decisions is often difficult to understand—even by the people who develop them. This lack of clarity can undermine trust in AI systems. To address this, explainable AI (XAI) has emerged as a solution, providing insights into how AI models arrive at their conclusions. Building trust through explainable AI is essential for its adoption in critical areas like healthcare, finance, and criminal justice, where the stakes are high.
What is Explainable AI?
Explainable AI refers to the development of AI models that can provide clear, understandable explanations of how they make decisions. Traditional AI, especially machine learning models such as deep learning networks, often operate as “black boxes,” where the internal workings are opaque. While these models can achieve high levels of accuracy, their complexity makes it difficult for users to interpret how they reached specific outcomes. This can be problematic in situations where decisions need to be justified, particularly in regulated industries like healthcare or financial services.
Explainable AI bridges this gap by making the decision-making process more transparent. XAI systems are designed to not only provide a result but also explain the reasoning behind it in a way that is accessible to non-experts. This enables users to understand and trust the model’s outputs, even when the algorithm itself is complex.
Why Trust in AI Matters
Trust is a fundamental requirement for AI adoption, particularly in fields where lives or livelihoods are impacted. For example, in healthcare, an AI system might recommend a course of treatment based on a patient’s medical data. If doctors and patients cannot understand why the system made that recommendation, they are less likely to trust the AI, no matter how accurate its predictions may be. The same applies to AI used in financial decision-making or legal judgments, where biases or mistakes could have severe consequences for individuals.
Building trust is not just about making AI more transparent but also about making it accountable. People need to believe that AI decisions are based on logical, unbiased factors and that those decisions can be questioned or audited when necessary. Explainable AI offers a path to achieving this trust by enabling stakeholders to scrutinize the underlying processes.
Key Benefits of Explainable AI
Explainable AI brings several key benefits, particularly in terms of fostering trust and ensuring responsible use of AI technologies.
Transparency
The most immediate benefit of explainable AI is its ability to make AI systems more transparent. Instead of simply providing an outcome, XAI systems break down the factors that led to that outcome. For example, if an AI model denies a loan application, explainable AI can reveal the key variables that influenced the decision, such as credit score, income level, or payment history. This transparency allows users to understand and question the AI’s reasoning, reducing the fear of arbitrary or biased decisions.
Accountability
AI systems, like humans, can make mistakes. Without a way to understand why an AI made a certain decision, it’s difficult to hold anyone accountable. Explainable AI introduces a level of accountability by revealing the logic behind decisions, enabling organizations to address errors or biases in the model. In regulated industries, this is particularly important because businesses need to ensure compliance with laws and regulations. With explainable AI, organizations can more easily demonstrate how their AI systems meet legal and ethical standards.
Fairness and Bias Mitigation
One of the most pressing concerns with AI is the risk of bias. AI models are trained on large datasets, and if those datasets contain biased information, the AI may perpetuate or even amplify those biases. Explainable AI helps mitigate this risk by providing insights into how decisions are made. For example, if a hiring algorithm disproportionately rejects candidates from certain demographic groups, explainable AI can help identify the problematic variables or patterns leading to those decisions. This allows organizations to adjust their models, promoting fairness and reducing discrimination.
Trust in AI-Driven Decisions
In sectors like healthcare, finance, and law enforcement, explainable AI can significantly improve trust. Consider the example of AI being used to predict whether a patient is at risk of developing a particular disease. If the model simply outputs “high risk” without explaining why, doctors and patients may question its reliability. However, if the AI can explain that its decision is based on specific factors like family history, lifestyle, or genetic markers, the reasoning becomes more transparent, and the recommendation more trustworthy.
Similarly, in criminal justice, AI is increasingly being used to assess the likelihood of reoffending. Without transparency, the use of such AI tools has been controversial, as hidden biases can lead to unfair sentencing. Explainable AI can provide clarity on how these assessments are made, helping legal professionals and the public understand and trust the system.
Implementing Explainable AI in Different Sectors
Different industries face unique challenges when it comes to implementing explainable AI. While the goal of enhancing trust remains consistent, the methods and priorities can vary based on the sector.
Healthcare
In healthcare, explainable AI is critical for ensuring that both patients and medical professionals can trust AI-driven diagnoses and treatment plans. AI systems that analyze medical data—such as diagnostic imaging or genomic information—can sometimes produce results that are counterintuitive to human doctors. By providing a clear explanation of how a particular diagnosis or treatment recommendation was made, XAI can foster collaboration between doctors and AI systems, leading to better patient outcomes.
For example, an AI model might flag early signs of a disease based on subtle patterns in medical imaging that a human radiologist could miss. Explainable AI can help the radiologist understand which areas of the image the AI focused on, providing a more comprehensive and trustworthy diagnosis.
Finance
In finance, explainable AI is crucial for ensuring that decisions regarding loans, investments, and credit assessments are fair and transparent. Financial institutions are subject to strict regulations, and they must be able to explain how decisions are made, especially when customers are denied loans or face increased interest rates. Explainable AI allows these institutions to break down complex decision-making processes into understandable components, ensuring compliance and building customer trust.
For example, if an AI-driven credit scoring model rejects a loan application, explainable AI can clarify which factors—such as income level, credit history, or debt-to-income ratio—contributed most to the decision. This transparency can help customers understand what they need to improve to qualify for future credit.
Law Enforcement and Criminal Justice
The use of AI in law enforcement has sparked significant ethical debates, particularly around bias and fairness. Explainable AI can address these concerns by ensuring that AI-driven decisions, such as predictive policing or risk assessments for bail or parole, are transparent and justifiable. By explaining the factors that led to a particular recommendation, XAI can help mitigate concerns about bias and ensure that AI is used responsibly in the justice system.
For instance, if an AI model predicts that an individual is at high risk of reoffending, explainable AI can reveal whether the decision was based on prior criminal behavior, socioeconomic factors, or other variables. This transparency is vital for ensuring that AI-driven decisions are fair and just.
Challenges of Explainable AI
While explainable AI offers significant benefits, implementing it comes with challenges. One of the biggest hurdles is balancing accuracy with interpretability. Many of the most accurate AI models, such as deep learning networks, are inherently complex, making it difficult to provide simple explanations for their decisions. In some cases, simpler models like decision trees or linear regressions may be easier to explain, but they may not perform as well in terms of accuracy.
Another challenge is ensuring that the explanations provided by XAI are truly understandable to the people using them. A technical explanation may satisfy AI experts, but it could confuse users without a background in data science. To build trust, AI explanations need to be tailored to different audiences, ensuring that they are both accurate and comprehensible.
A Future Built on Transparent AI
As AI continues to shape our world, its success will depend on the trust it can build with users. Explainable AI represents a critical step in ensuring that AI systems are transparent, accountable, and fair. By providing clear and understandable reasoning behind AI-driven decisions, we can foster greater trust in these systems, enabling them to be used responsibly in sectors that directly impact people’s lives.
Explainable AI is more than just a technical feature—it is a commitment to ethical AI development and a promise that AI will serve humanity in a way that is transparent, fair, and accountable. As organizations across industries continue to integrate AI into their operations, explainability will be essential for building the trust needed to fully unlock AI’s potential for good.