Cracking the Bias Code: Building Fairer Language Models, One Word at a Time
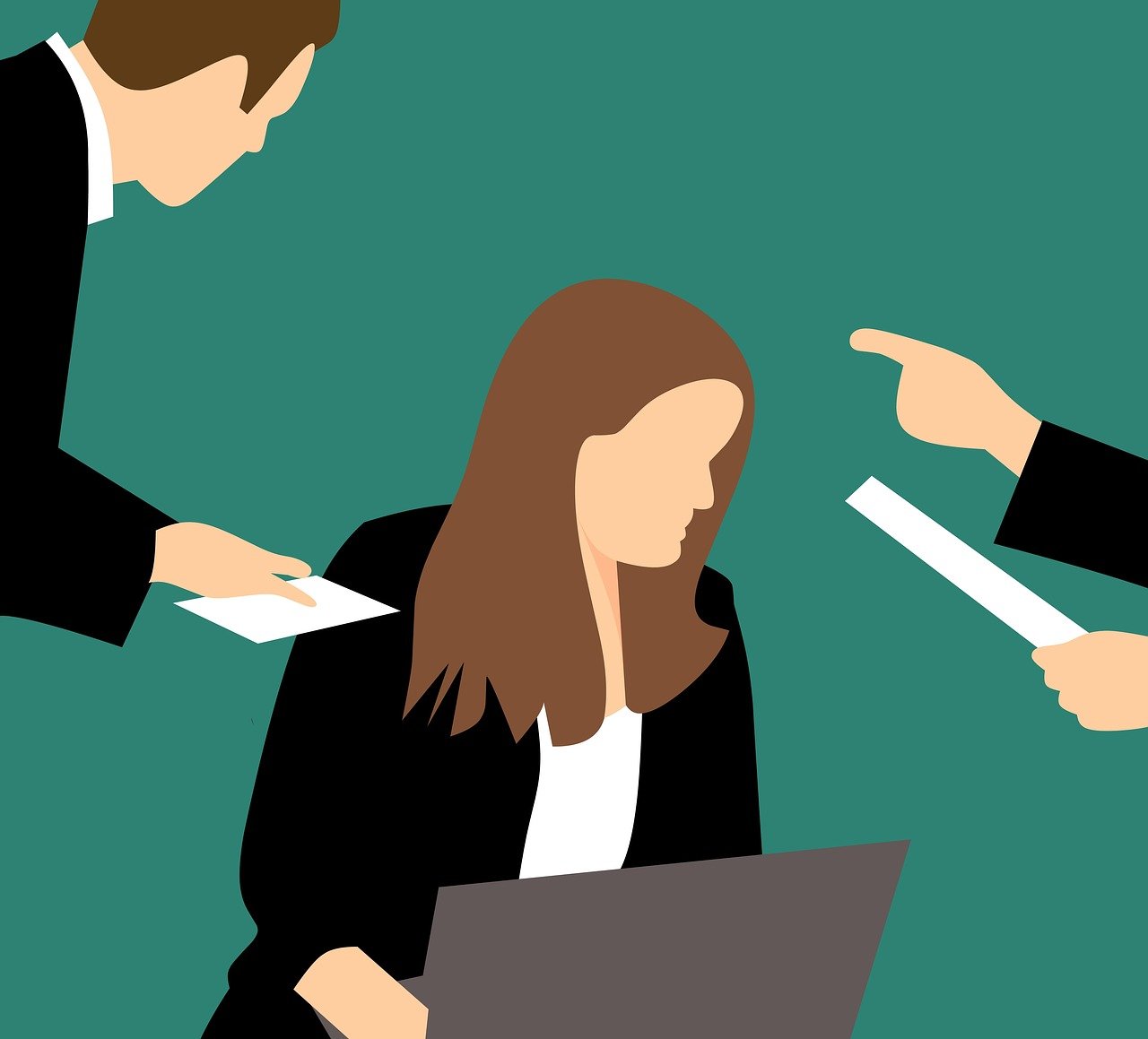
Your friendly AI assistant casually cracks a sexist joke or perpetuates harmful stereotypes. Unsettling, right? Unfortunately, this dystopian reality isn’t science fiction; it’s the potential pitfall of language models (LMs), powerful tools that mimic human speech and also inherit and amplify biases from their training data. But fret not, language enthusiasts! Let’s embark on a quest to understand how bias infects LMs and explore strategies to build equitable, inclusive language machines.
The Bias Echo Chamber: Where Does It Begin?
LMs act as sponges, meticulously absorbing the information they’re fed. Text datasets, their lifeblood, often reflect societal biases. From historical texts laced with gender stereotypes to social media brimming with discriminatory language, these biases become ingrained in the model’s understanding of the world. It’s like a game of telephone gone wrong – the bias gets amplified with each iteration, leading to potentially harmful outputs.
When Words Have Consequences
Consider Tay, Microsoft’s chatbot that learned racist and offensive language within 24 hours. The AI image captioning tool that labeled black people as criminals more often than white people. These are stark reminders of the real-world consequences of unchecked bias in LMs. From perpetuating stereotypes to reinforcing societal inequalities, biased language models can have a negative impact on individuals and communities.
Breaking the Cycle: Techniques for Mitigating Bias
We’re not stuck in this echo chamber. Researchers and developers are actively exploring various strategies to neutralize bias in LMs:
Data Detoxification: Imagine meticulously cleaning your room. Data detoxification does the same for training data. Techniques like identifying and removing biased language, diversifying datasets with inclusive content, and employing data augmentation with counterfactual scenarios (“what if this character had a different race?”) help create fairer training grounds for LMs.
Algorithmic Awareness: Think of it as teaching LMs about bias itself. Adversarial training, which exposes the model to deliberately biased inputs, and counterfactual fairness approaches, which analyze model outputs across different hypothetical scenarios, help language models (LMs) recognize and resist bias. hypothetical scenarios, can help language models (LMs) recognize and resist bias.patterns.
Fairness Metrics: Just like measuring success in other areas, quantifying bias is crucial. Researchers are actively developing metrics, such as fairness measures based on protected characteristics and differential privacy techniques, which safeguard individual privacy while analyzing model outputs. As a result, these advancements allow for more objective evaluation and enable targeted mitigation strategies.
Beyond Technology: Showcasing Inclusive AI Projects
While technology plays a crucial role, addressing bias in LMs goes beyond just tools and techniques. Let’s look at some inspiring AI projects promoting inclusivity:
Project Include: This Google AI initiative focuses on developing fairness-aware machine learning techniques like counterfactual fairness and fostering responsible AI development practices.
Algorithmic Justice League: This organization works to identify and mitigate bias in algorithms, advocating for fairer and more equitable AI systems.
Megatron-Turing NLG: This large language model, developed by NVIDIA and partners, utilizes data detoxification, fairness metrics, and algorithmic awareness techniques like adversarial training to address potential biases in its outputs.
Journalism: AI-powered tools are being used to analyze large datasets and identify potential biases in news coverage, promoting fairer and more inclusive reporting.
Finance: LMs are being developed to analyze financial data and identify potential discrimination against marginalized groups, fostering fairer lending practices.
Ethical Considerations: When Does Convenience Meet Responsibility?
The use of LMs in sensitive domains like education or healthcare raises critical ethical questions. Consider the potential biases in AI-powered grading systems or the implications of biased language models used in medical diagnosis. Open discussions and transparent development practices are crucial to ensure the responsible and ethical application of LMs in these areas. This can be accomplished by using robust ethical frameworks like the Montreal Declaration for Responsible AI guiding development and deployment.
The benefits of LMs in education, like personalized learning recommendations, or in healthcare, like analyzing medical records for faster diagnoses, must be weighed against the potential risks of bias amplification. Building truly inclusive LMs requires a multi-pronged approach where technology, ethical frameworks, and societal awareness work hand-in-hand.