Data Privacy in the AI Era: Balancing Insight and Trust
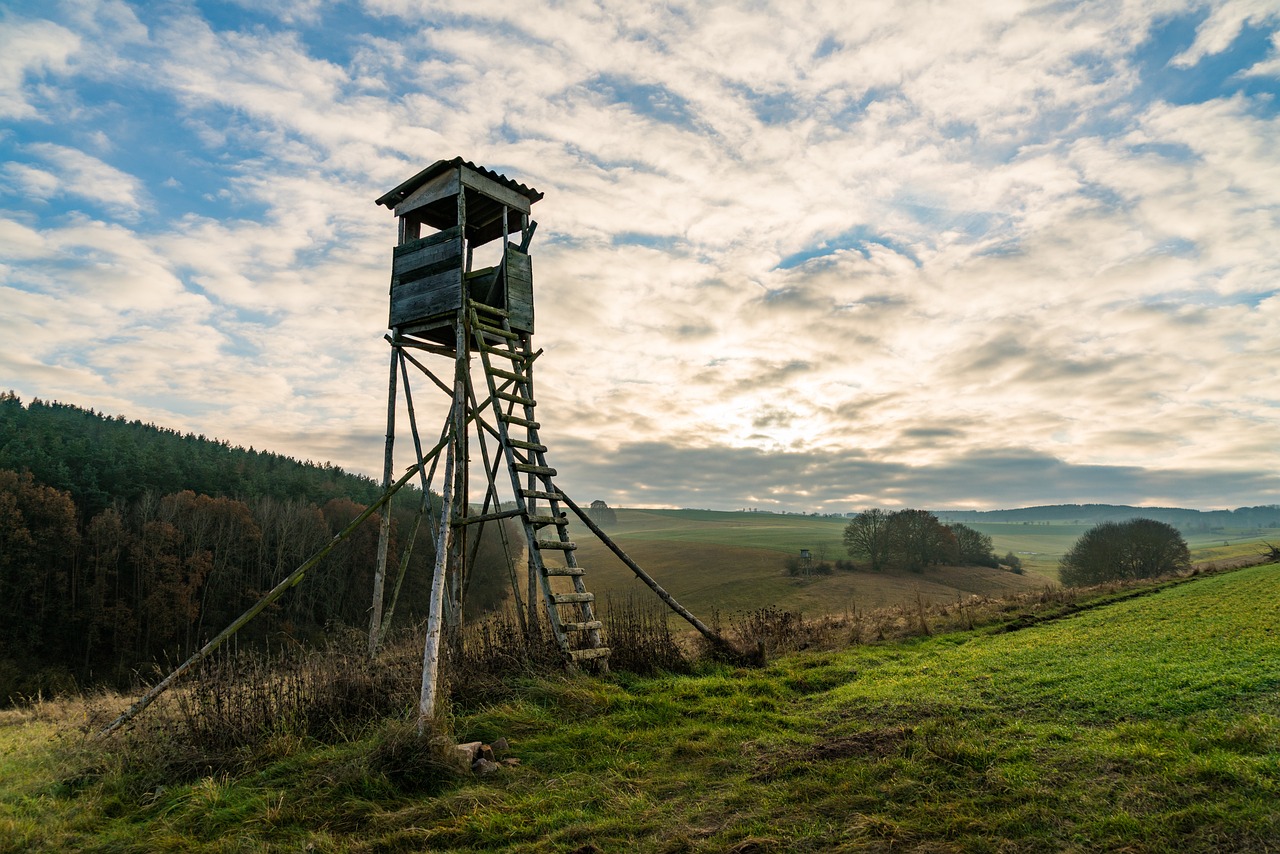
As artificial intelligence (AI) continues to reshape industries and societies, the volume of data being collected, analyzed, and processed has skyrocketed. From personalized recommendations on streaming platforms to predictive healthcare models, AI-driven insights offer immense value. However, with great power comes great responsibility—data privacy has become one of the most critical concerns of our time. In the AI era, where data fuels innovation, organizations face a unique challenge: how to balance the demand for insights while maintaining trust and safeguarding individual privacy.
This article delves into the complexities of data privacy in the AI era, examining the ethical, technical, and regulatory dimensions of protecting personal data while leveraging AI’s capabilities.
The Growing Importance of Data in AI
AI systems thrive on data. The more data they have, the better they can learn, adapt, and generate valuable insights. This makes data the foundation of AI innovation, from machine learning algorithms that identify patterns in user behavior to deep learning models that can predict diseases based on medical records.
In many ways, data is the new oil—a vital resource that powers modern digital economies. Companies collect vast amounts of data to improve services, optimize operations, and create personalized experiences. Governments use data to enhance public services, and healthcare providers rely on patient data to drive breakthroughs in medicine.
However, with the explosion of data comes a darker side: the potential for misuse, breaches, and erosion of trust. Personal data—such as health records, financial information, and even daily browsing habits—can reveal intimate details about an individual’s life. Without proper safeguards, this data can be exploited, leading to privacy violations, identity theft, and discrimination.
This tension between harnessing data for AI-driven insights and protecting privacy is at the heart of the current debate on data privacy in the AI era.
Why Data Privacy Matters in the AI Era
Data privacy refers to the practices and measures used to protect personal information from unauthorized access, misuse, or disclosure. In the context of AI, the need for privacy is heightened due to the sheer volume and sensitivity of data that AI systems process.
Several factors underscore the importance of data privacy in AI:
1. Sensitive Data Exposure
AI models often rely on vast datasets that include sensitive personal information—such as biometric data, medical histories, and financial records. If this data is mishandled or falls into the wrong hands, it can have severe consequences for individuals. Breaches of healthcare data, for example, could expose medical conditions, while leaks of financial data can lead to fraud.
2. Lack of Transparency in AI Decision-Making
One of the key concerns about AI systems is their lack of transparency. Complex machine learning algorithms, especially in deep learning, operate as “black boxes,” meaning that even their developers cannot always explain how decisions are made. This opacity can create ethical issues when AI makes decisions that impact people’s lives, such as hiring, credit scoring, or medical treatment recommendations. Without transparency, it becomes difficult to ensure that data is being used ethically and legally.
3. Trust Erosion
Public trust is essential for the adoption of AI technologies. When individuals feel that their data is being collected without consent or used in ways they did not anticipate, it erodes trust. Trust is fragile—one high-profile data breach can damage a company’s reputation and lead to significant legal and financial repercussions. Maintaining strong data privacy practices is key to ensuring that individuals remain confident in sharing their data with AI-driven systems.
4. Ethical Implications
AI systems, when fed with biased or incomplete data, can perpetuate discrimination and unfair treatment. For example, facial recognition algorithms have been shown to have higher error rates when identifying people with darker skin tones, leading to discriminatory outcomes. Ethical AI requires that data privacy is upheld not only to protect individuals but also to prevent the misuse of data that could result in biased AI outcomes.
5. Legal and Regulatory Pressures
In response to growing concerns around privacy, governments and regulatory bodies around the world have enacted stringent data protection laws. The European Union’s General Data Protection Regulation (GDPR), for instance, has set a global standard for data privacy, requiring organizations to be transparent about how they collect, process, and use personal data. Failure to comply with these regulations can lead to substantial fines and penalties, making data privacy not just an ethical issue, but a legal one as well.
Balancing Insight and Privacy: The AI Dilemma
The challenge of balancing the demand for AI insights with the need for data privacy is multifaceted. On the one hand, AI systems require access to large and diverse datasets to generate accurate, meaningful insights. On the other hand, individuals have the right to control how their personal information is collected, used, and shared.
Here are some of the key issues that organizations must navigate to achieve this balance:
1. Data Minimization
One principle of data privacy is data minimization—the idea that organizations should collect only the data they absolutely need to achieve a specific purpose. In practice, however, AI projects often operate under the assumption that “more data is better.” The more data an AI system can access, the more accurate and nuanced its predictions can be.
To balance these needs, organizations should adopt a more thoughtful approach to data collection. Rather than gathering massive amounts of data indiscriminately, AI developers should focus on collecting the minimum amount of data required to achieve their goals while ensuring that data is anonymized whenever possible.
2. Anonymization and De-identification
One of the most effective ways to protect privacy while allowing AI systems to analyze data is through anonymization and de-identification. Anonymization involves removing personally identifiable information (PII) from datasets so that individual identities cannot be traced. De-identification similarly removes or masks PII but may allow for the data to be re-identified under certain conditions.
Anonymizing data is particularly important in industries like healthcare, where sensitive patient data is used to train AI models. However, even anonymized data can sometimes be re-identified through sophisticated techniques, posing a challenge to privacy protection. Therefore, ongoing research into more robust anonymization techniques is critical for maintaining privacy in AI applications.
3. Differential Privacy
To further protect individual privacy, AI developers are turning to advanced techniques such as differential privacy. Differential privacy introduces statistical noise into datasets, ensuring that individual records cannot be isolated or identified while still allowing AI algorithms to learn from the data.
Differential privacy is particularly valuable for organizations that rely on large datasets to build AI models. By using this technique, companies can continue to extract insights from data without compromising the privacy of individuals within the dataset.
4. Federated Learning
Another promising approach to balancing AI insights with privacy is federated learning. In traditional AI development, data from multiple sources is pooled into a central location for analysis. In contrast, federated learning allows AI models to be trained across decentralized devices or servers, meaning that the data never leaves its original source. Instead of sharing raw data, devices share only the model updates based on the data.
This approach significantly reduces privacy risks, as the raw data remains securely on local devices, while still enabling the AI system to learn and improve from distributed datasets. Federated learning is already being used in applications like mobile devices, where AI models can learn from user interactions without transmitting personal data to central servers.
5. Transparent AI Systems and Accountability
To build trust and protect privacy, organizations must prioritize transparency in their AI systems. This includes being clear about what data is being collected, how it is being used, and for what purpose. It also involves developing AI systems that are explainable—meaning that users and regulators can understand how decisions are being made.
Transparency goes hand in hand with accountability. Organizations should establish clear protocols for managing data privacy and assign responsibility for overseeing data protection practices. This may involve appointing a Data Protection Officer (DPO) or implementing regular audits to ensure compliance with data privacy regulations.
The Role of Regulations in Data Privacy and AI
As AI continues to advance, governments around the world are implementing stricter data privacy regulations to ensure that individuals’ rights are protected. These regulations aim to strike a balance between enabling innovation and safeguarding privacy.
1. General Data Protection Regulation (GDPR)
The European Union’s GDPR is perhaps the most comprehensive data privacy law to date. It gives individuals greater control over their personal data, including the right to access, correct, and delete their data. For AI systems, GDPR requires organizations to obtain clear consent for data processing and to ensure that AI decision-making processes are transparent and explainable.
The GDPR’s impact extends beyond Europe, influencing data privacy laws globally and setting a high standard for privacy protection.
2. California Consumer Privacy Act (CCPA)
In the United States, the California Consumer Privacy Act (CCPA) is a landmark law that gives California residents more control over their personal data. Under CCPA, individuals have the right to know what data is being collected, opt out of the sale of their data, and request the deletion of their data.
The CPA is an important step toward stronger data privacy protections in the U.S., and other states are following California’s lead by enacting similar legislation.
3. Future Regulations
As AI technology continues to evolve, we can expect to see more regulations specifically tailored to the ethical use of AI. These future regulations may focus on preventing bias in AI systems, ensuring algorithmic transparency, and protecting against the misuse of personal data in AI-driven decision-making.
Building Trust in the AI Era
Ultimately, the success of AI hinges on trust. Organizations that prioritize data privacy and transparency will be better positioned to earn the trust of their users, customers, and partners. By adopting ethical data practices and complying with privacy regulations, companies can strike the right balance between generating AI-driven insights and protecting individual rights.
Key steps for building trust in AI include:
- Clear Communication: Be upfront with users about how their data is being collected and used.
- Opt-in Policies: Obtain explicit consent before using personal data for AI purposes.
- Data Security: Invest in robust cybersecurity measures to protect data from breaches.
- Explainability: Develop AI systems that provide clear, understandable explanations for decisions.
The Path Forward for Data Privacy and AI
As we continue to unlock the potential of AI, protecting data privacy will remain one of the most critical challenges of the digital age. The balance between insight and trust is delicate, but by prioritizing ethical data practices, adopting privacy-enhancing technologies, and adhering to regulatory frameworks, organizations can navigate this complex landscape.
The future of AI will depend not only on its ability to generate powerful insights but also on its capacity to do so while upholding the values of trust, transparency, and respect for privacy. As the AI era progresses, organizations that successfully balance these priorities will lead the way in creating a digital ecosystem where both innovation and individual rights can thrive.