Edge AI: Intelligence at the Periphery
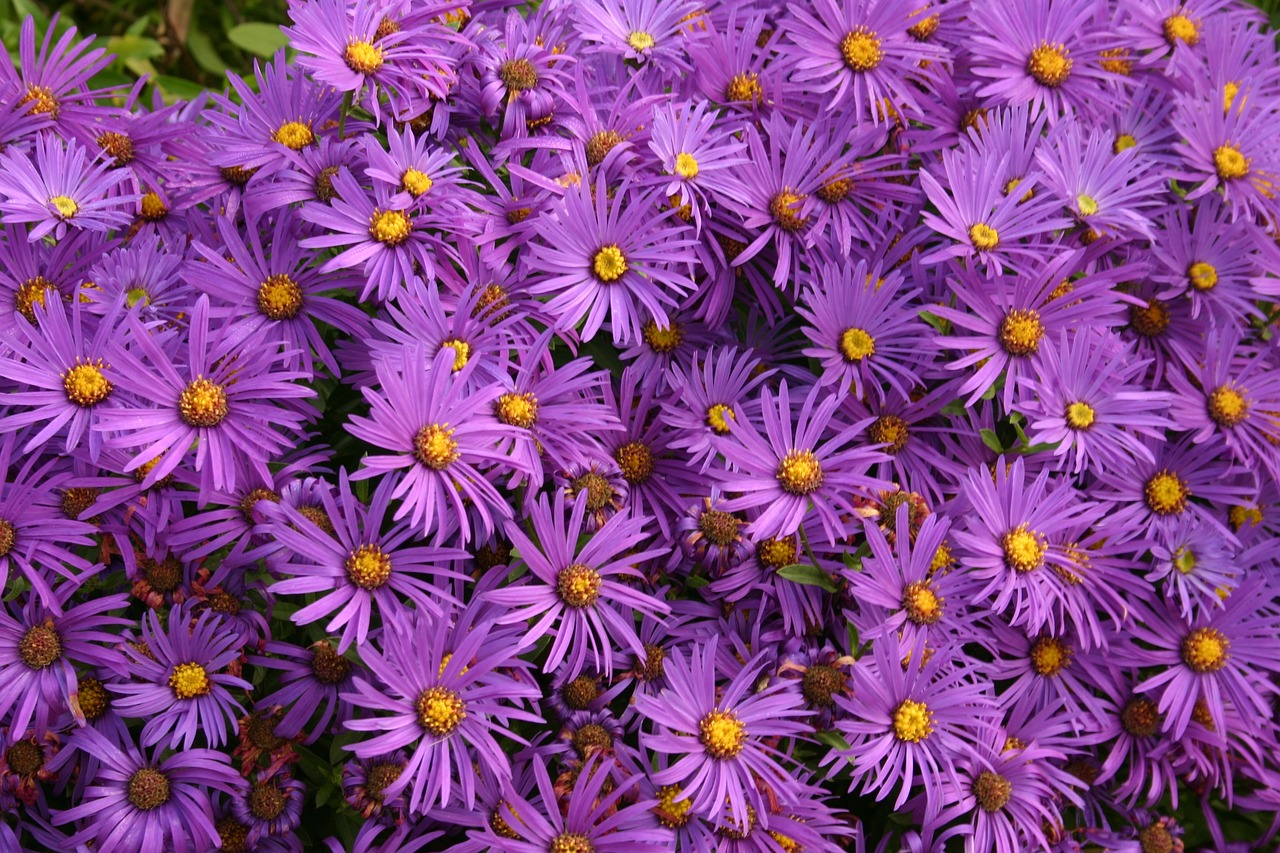
As the demand for real-time data processing grows, Edge AI is emerging as a game-changer in industries ranging from healthcare to manufacturing. Unlike traditional AI, which often relies on cloud computing for data processing, Edge AI brings intelligence directly to the device or network edge. This shift has profound implications for latency, security, and energy efficiency. In this article, we’ll explore what Edge AI is, how it works, its benefits, and why it represents the next frontier in artificial intelligence.
What is Edge AI?
Edge AI refers to the deployment of artificial intelligence models directly on edge devices—such as smartphones, IoT sensors, or even autonomous vehicles—without needing to send data back to centralized servers for processing. It operates at or near the source of data generation, enabling faster decision-making and reducing reliance on cloud-based data centers.
Edge AI combines the power of machine learning and data analytics with localized computing power, allowing devices to make intelligent decisions independently. This is particularly beneficial in situations where real-time responses are critical or when constant cloud connectivity is impractical.
How Edge AI Works
The process of Edge AI involves deploying pre-trained AI models on edge devices. These models are developed and refined using vast amounts of data in centralized locations—often using cloud computing—and then optimized for performance on smaller, localized hardware.
Here’s a step-by-step look at how Edge AI functions:
- Data Generation: Edge devices, such as sensors or cameras, continuously generate data.
- Model Deployment: Pre-trained AI models are deployed on these devices, allowing them to process data locally.
- Real-Time Decision Making: Once the AI models are embedded in the device, they can analyze data in real time and make decisions or trigger actions, all without sending the data to the cloud.
- Occasional Syncing with Cloud: While the primary processing happens at the edge, some devices may occasionally sync with the cloud for updates, retraining of models, or to share insights for broader analysis.
This decentralized model of computation greatly reduces the need for constant connectivity, which is essential in environments where cloud access is limited or where data privacy concerns are paramount.
Key Benefits of Edge AI
Edge AI offers several key advantages, particularly in applications where speed, security, and scalability are crucial. Below, we explore some of the most significant benefits:
1. Real-Time Processing and Low Latency
One of the most compelling benefits of Edge AI is its ability to process data in real time. For many industries, from autonomous vehicles to healthcare monitoring systems, delays caused by sending data back to the cloud for processing can be unacceptable. Edge AI dramatically reduces this latency by bringing computation closer to the data source. For example, in autonomous driving, the ability to make split-second decisions could mean the difference between avoiding an accident and causing one.
2. Enhanced Data Privacy and Security
Data privacy is a growing concern, especially as more devices become interconnected in the Internet of Things (IoT) ecosystem. Edge AI helps address these concerns by keeping data local to the device. Since data doesn’t need to be transferred to a cloud server for processing, the risk of sensitive information being intercepted or compromised is significantly reduced. This localized approach is particularly advantageous in healthcare, finance, and government sectors, where data security is paramount.
3. Reduced Bandwidth and Energy Consumption
Cloud computing requires a significant amount of data to be sent back and forth between devices and servers, consuming considerable bandwidth and energy in the process. By processing data locally, Edge AI minimizes the need for constant data transmission, leading to more efficient use of bandwidth. This reduction in data flow also results in lower energy consumption, which is particularly beneficial in large-scale IoT deployments where multiple devices need to operate efficiently over long periods.
4. Scalability in IoT Applications
As IoT devices continue to proliferate, the sheer volume of data they generate can overwhelm centralized cloud infrastructures. Edge AI offers a scalable solution by enabling devices to process much of this data locally. This decentralized approach reduces the burden on cloud networks and allows IoT ecosystems to grow without causing bottlenecks or delays in data processing.
5. Offline Functionality
Not every environment has reliable internet connectivity. Whether it’s a remote factory, an underground mine, or an autonomous vehicle in a rural area, many edge devices operate in locations where cloud connectivity is inconsistent or unavailable. Edge AI allows these devices to continue functioning independently, making real-time decisions even when they’re offline. This makes Edge AI particularly attractive for industries that operate in remote or challenging environments.
Edge AI Use Cases
The adoption of Edge AI is growing rapidly, with numerous industries finding innovative applications for this technology. Here are a few key areas where Edge AI is making an impact:
1. Autonomous Vehicles
Autonomous cars need to process vast amounts of sensor data in real time to make safe driving decisions. Cloud-based processing would introduce too much latency, making it unsuitable for such critical tasks. Edge AI enables vehicles to process data from cameras, LIDAR, and radar locally, allowing them to react instantly to changes in their environment.
2. Healthcare
Wearable devices and remote health monitoring systems generate a wealth of data, which can be processed using Edge AI to provide real-time insights. For example, a smart medical device equipped with AI can monitor a patient’s vital signs and detect anomalies like irregular heartbeats. These insights can be acted upon immediately without needing to wait for cloud processing.
3. Smart Manufacturing
In the manufacturing sector, Edge AI is used to monitor machinery, detect anomalies, and predict maintenance needs. By processing sensor data directly on the factory floor, manufacturers can prevent equipment failures and reduce downtime. This local processing ensures that decisions are made quickly, avoiding costly delays that could arise from cloud-based data processing.
4. Retail
Retailers are beginning to use Edge AI for personalized in-store experiences. Smart cameras and sensors analyze customer behavior in real time, allowing businesses to make targeted product recommendations, optimize store layouts, and manage inventory levels more effectively.
5. Smart Cities
Cities around the world are deploying IoT devices to improve public services such as traffic management, waste collection, and energy distribution. Edge AI enables these systems to operate efficiently by processing data locally, reducing the need for constant cloud communication and ensuring that decisions can be made quickly, even during network outages.
Challenges in Deploying Edge AI
While Edge AI offers numerous benefits, it also presents unique challenges that businesses must navigate:
1. Hardware Limitations
Edge devices are often constrained in terms of processing power, memory, and energy consumption. Unlike cloud servers, which can handle heavy computational loads, edge devices need to operate efficiently with limited resources. This requires careful optimization of AI models to ensure they can run effectively on smaller devices.
2. Model Deployment and Maintenance
Deploying AI models on edge devices and keeping them updated can be a complex process. Once deployed, these models need to be maintained, which often requires periodic updates or retraining. Ensuring that all edge devices receive these updates—particularly when they operate in remote or offline environments—can pose logistical challenges.
3. Data Fragmentation
With data processing occurring across multiple edge devices, organizations must find ways to manage and integrate data from various sources. Ensuring that all devices work cohesively and that data is harmonized for broader analysis is critical for Edge AI success.
The Future of Edge AI
As advancements in AI models, hardware, and connectivity continue, Edge AI is poised to become even more integral to a variety of industries. The proliferation of 5G networks, for example, will enhance the ability of edge devices to communicate with each other and with cloud servers, enabling more complex AI applications at the edge.
Furthermore, we can expect greater synergy between cloud and edge computing, where the cloud continues to handle large-scale data processing and model training, while edge devices execute real-time decision-making. This hybrid approach will likely dominate the future AI landscape.
Intelligent Solutions at the Edge
Edge AI is transforming how businesses operate by decentralizing data processing and bringing intelligence to the periphery. Its ability to provide real-time insights, enhance data security, reduce costs, and enable offline functionality makes it an invaluable tool in industries ranging from healthcare to manufacturing.
As the technology continues to evolve, Edge AI will play a pivotal role in the broader AI ecosystem, empowering businesses to operate more efficiently and securely while adapting to the ever-growing demands of data-driven decision-making.