Encoding Social Good: Can We Teach AI to Be Fair?
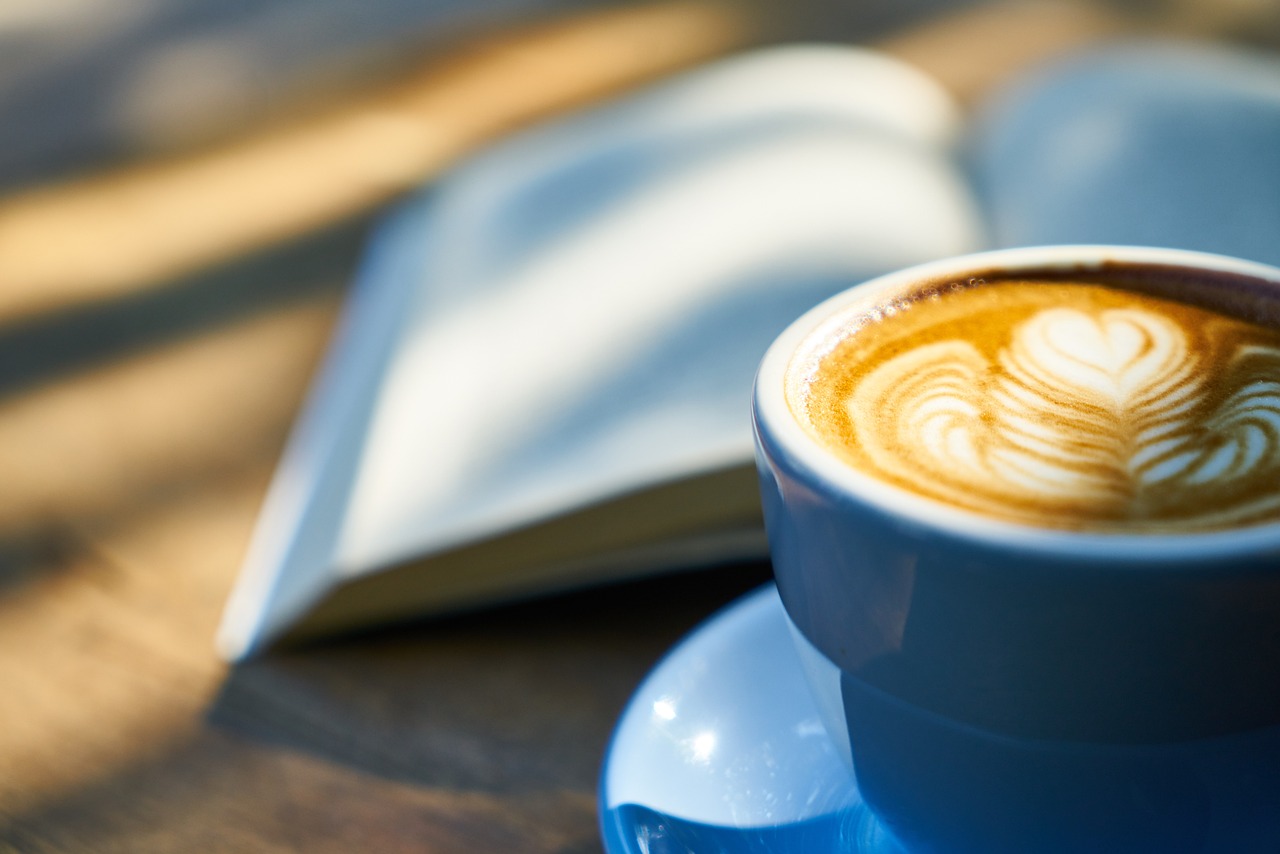
As artificial intelligence (AI) systems become more prevalent in our daily lives, questions about their fairness and potential biases have moved to the forefront of tech discussions. From hiring processes to loan approvals, AI now plays a significant role in decisions that can profoundly impact people’s lives. But can we ensure these systems make choices that align with our societal values of fairness and equality?
The Challenge of Defining Fairness
One of the primary hurdles in creating fair AI systems is the complexity of defining fairness itself. What seems fair in one context may not be in another, and different cultures and individuals often have varying perspectives on what constitutes equitable treatment.
Research suggests that there are multiple, sometimes conflicting, definitions of fairness in machine learning. These can include demographic parity, equal opportunity, and individual fairness, among others. Each definition addresses different aspects of fairness and may be more appropriate in certain scenarios.
Tackling Bias in AI Systems
AI systems learn from historical data, which often contains human biases. This can lead to the perpetuation and even amplification of existing societal inequalities if not carefully managed.
To address this issue, many companies are implementing bias detection and mitigation strategies. These may involve diverse data collection methods, careful feature selection, and the use of algorithms designed to promote fairness.
However, completely eliminating bias remains a significant challenge. Some experts argue that the goal should be to make AI systems more transparent and accountable rather than striving for perfect neutrality.
The Role of Diverse Teams
Creating fair AI systems requires input from a wide range of perspectives. Studies indicate that diverse teams are better equipped to identify potential biases and develop more inclusive solutions.
Many tech companies are now prioritizing diversity in their AI development teams. This includes not just gender and ethnic diversity, but also diversity in academic backgrounds, bringing together computer scientists, ethicists, social scientists, and legal experts.
Looking Ahead: The Future of Fair AI
As AI continues to advance, the quest for fairness will likely remain a central concern. Ongoing research is exploring new methods for evaluating and improving the fairness of AI systems, including advanced testing frameworks and novel algorithmic approaches.
The development of fair AI also has significant business implications. Companies that can demonstrate the fairness and trustworthiness of their AI systems may gain a competitive edge, particularly in sensitive areas like healthcare and finance.
Ultimately, creating truly fair AI systems will require ongoing collaboration between technologists, policymakers, and society at large. As we continue to integrate AI into critical decision-making processes, ensuring these systems align with our values of fairness and equality will be essential for building public trust and maximizing the benefits of this powerful technology.