From Data Swamps to Data Lakes: AI-Driven Data Quality
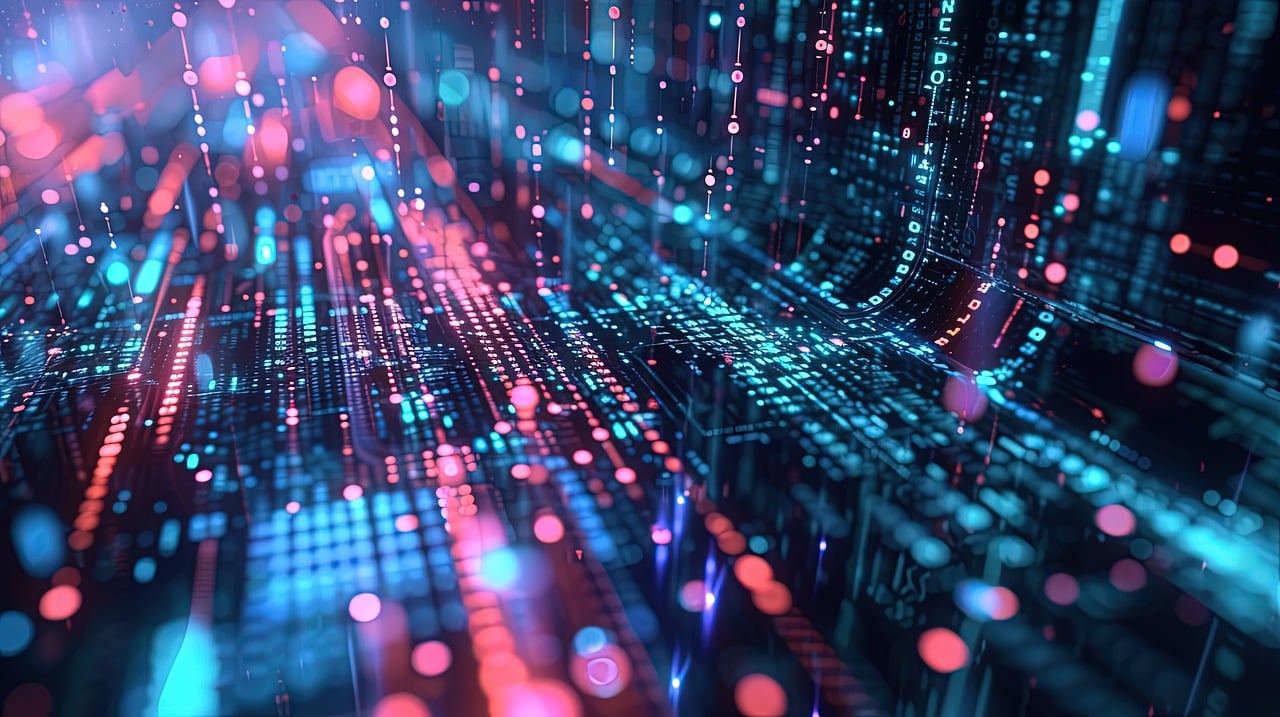
As businesses become increasingly data-driven, the challenge of managing vast amounts of information has grown exponentially. Data has the potential to be one of the most valuable assets for any organization, but only if it’s properly structured, maintained, and accessible. Unfortunately, many organizations face the issue of data swamps—unorganized, unusable collections of information that can lead to poor decision-making. To combat this, AI-driven approaches are transforming these data swamps into data lakes, providing not just storage but also enhanced data quality, accessibility, and actionable insights.
What Is a Data Swamp?
A data swamp is a mismanaged data repository where information is collected without adequate oversight, leading to disorganized, low-quality data. It’s a term used to describe what happens when data lakes—repositories designed to hold massive amounts of structured and unstructured data—become ungoverned, creating significant challenges for data retrieval, analysis, and management.
In a data swamp, data may be incomplete, duplicated, outdated, or lacking proper metadata, making it difficult for data scientists, analysts, and business teams to find valuable insights. Instead of empowering decision-making, data swamps often lead to confusion and inefficiencies. The core problem stems from a lack of data governance and poor data quality controls.
Without clear structures or validation processes in place, organizations can fall into the trap of storing more and more data without ensuring its accuracy or relevance. The result? Information overload, where decision-makers are unsure which data to trust.
Transforming Data Swamps into Data Lakes
To turn a data swamp into a data lake—a well-organized, highly functional data repository—organizations must focus on data quality, governance, and usability. A data lake stores raw, structured, semi-structured, and unstructured data in a way that allows it to be easily searched, retrieved, and analyzed. The key difference between a data swamp and a data lake is the application of AI-driven processes to ensure data integrity, consistency, and accessibility.
AI can dramatically improve data management by automating the cleaning, organizing, and categorizing of large datasets. These processes ensure that data in a data lake is accurate, up-to-date, and relevant, unlocking its potential for generating valuable insights. AI’s ability to rapidly process and analyze vast amounts of information provides the foundation for creating a data lake that serves as a reliable source of truth for an organization.
The Role of AI in Ensuring Data Quality
AI-driven tools play a pivotal role in enhancing data quality by automating critical processes such as cleaning, validation, and governance. Here are some ways AI is helping organizations maintain high-quality data in their data lakes:
1. Automated Data Cleansing
One of the most time-consuming tasks in data management is cleansing—removing inaccuracies, duplicates, and irrelevant information from datasets. Manual data cleaning can be slow, error-prone, and difficult to scale as data volumes grow. AI-powered tools can automate this process, identifying outliers, filling missing values, and removing redundant records in real-time. Machine learning algorithms can learn from patterns in the data, continuously improving the quality of the cleansing process.
For example, IBM Watson uses AI-driven data cleansing tools to automatically detect and correct inconsistencies in datasets. By applying pattern recognition and predictive analytics, these tools can rapidly clean massive amounts of data, ensuring higher levels of accuracy for future analyses.
2. Data Classification and Tagging
Data lakes often hold a wide range of unstructured data—such as images, videos, and text documents—that need to be categorized and tagged for easier retrieval. AI tools can automate the classification and tagging process by analyzing the content and context of each data element, applying appropriate metadata that makes the data searchable and discoverable.
Amazon Web Services (AWS) offers Amazon Macie, an AI-powered tool that automatically discovers, classifies, and protects sensitive data stored in data lakes. Macie uses machine learning to classify data such as personal identifiers, financial information, or intellectual property, ensuring that sensitive data is tagged and protected while enhancing searchability for analysis.
3. Data Deduplication and Integrity Checks
Data duplication is a major issue that can degrade the quality of information stored in a data lake, leading to inaccurate analysis and decision-making. AI-powered data deduplication tools scan for repeated records, eliminating unnecessary copies while preserving the most relevant and accurate information.
For example, Microsoft Azure’s Purview uses machine learning to conduct integrity checks and ensure that data is unique, accurate, and properly integrated across various systems. This AI-driven approach prevents the accumulation of duplicate or erroneous data in the repository, improving overall data quality and reducing storage costs.
4. Real-Time Data Monitoring
Data lakes are often dynamic, with new information constantly flowing in from multiple sources. Keeping track of changes and ensuring the integrity of this data in real-time can be challenging without the aid of AI. AI-driven data monitoring systems track and validate incoming data, flagging inconsistencies, anomalies, or missing values as soon as they occur.
By continuously analyzing streaming data, AI can ensure that only high-quality information is added to the data lake. Google Cloud’s Dataplex uses machine learning models to automate data quality checks and real-time monitoring, preventing the degradation of data quality over time.
Data Governance: AI’s Role in Managing Data at Scale
Another critical component of transforming a data swamp into a functional data lake is data governance. Data governance involves defining and implementing policies that ensure data accuracy, consistency, privacy, and security throughout its lifecycle. AI is essential for maintaining governance in large-scale data environments by automating the enforcement of rules and standards.
1. Data Lineage and Auditing
Data lineage tracks the origin and movement of data through the organization. It provides visibility into where the data came from, how it has been processed, and where it is being used. AI-powered data lineage tools automatically trace the flow of data, ensuring transparency and accountability.
Collibra, a data governance platform, uses AI to automate data lineage tracking across systems, allowing organizations to audit data usage, ensure compliance with regulations, and track changes over time. This helps prevent data swamps by maintaining clear records of how data is transformed, used, and stored.
2. Access Control and Security
AI can enhance data governance by enforcing access controls that protect sensitive data. AI-powered tools can monitor who is accessing specific datasets, flagging unauthorized access attempts or unusual activity. They also allow organizations to apply automated encryption and anonymization techniques to protect personal or sensitive information.
For instance, Snowflake’s data platform includes AI-driven security features that provide real-time monitoring and access control. By automating the process of data classification and access management, Snowflake ensures that sensitive data is protected while still being accessible to authorized users.
3. Compliance and Privacy Management
Data privacy regulations like GDPR and CCPA place strict requirements on how organizations handle and process personal data. AI tools can assist with compliance by automatically identifying and tagging personal data, ensuring that it’s managed according to regulatory requirements.
BigID, a privacy-focused AI platform, uses machine learning to identify and classify personal data across data lakes. It then applies appropriate privacy controls, ensuring that organizations can comply with global data regulations while maintaining data integrity and accessibility.
The Benefits of AI-Driven Data Lakes
Transforming a data swamp into a well-managed data lake provides numerous benefits for businesses, especially when AI is used to drive data quality improvements:
1. Enhanced Decision-Making
By ensuring that data is clean, accurate, and well-organized, AI-driven data lakes provide a reliable foundation for data analysis. Businesses can make better, faster decisions with high-quality data, leading to improved operational efficiency and more informed strategic planning.
2. Improved Scalability
AI automation allows data lakes to scale without sacrificing quality. As data volumes grow, AI-driven tools ensure that incoming data is validated, categorized, and deduplicated in real-time, preventing the data lake from turning back into a data swamp.
3. Cost Efficiency
AI-driven processes reduce the time and resources needed to manage and clean large datasets, leading to cost savings. By automating routine tasks like data cleansing and governance, organizations can focus more on analyzing and utilizing data, rather than managing it.
AI as the Key to Managing Data Quality
The transition from data swamps to data lakes is vital for organizations looking to maximize the value of their data assets. AI-driven data quality solutions ensure that data lakes remain organized, accessible, and actionable, turning overwhelming data sets into reliable sources of business intelligence. By applying AI to automate data cleansing, governance, and real-time monitoring, companies can unlock the full potential of their data while avoiding the pitfalls of unmanaged data storage.
As the volume and complexity of data continue to grow, AI will remain an essential tool for businesses striving to maintain data quality, optimize performance, and make informed, data-driven decisions.