The AI Adoption Roadmap: Welcoming In The Future
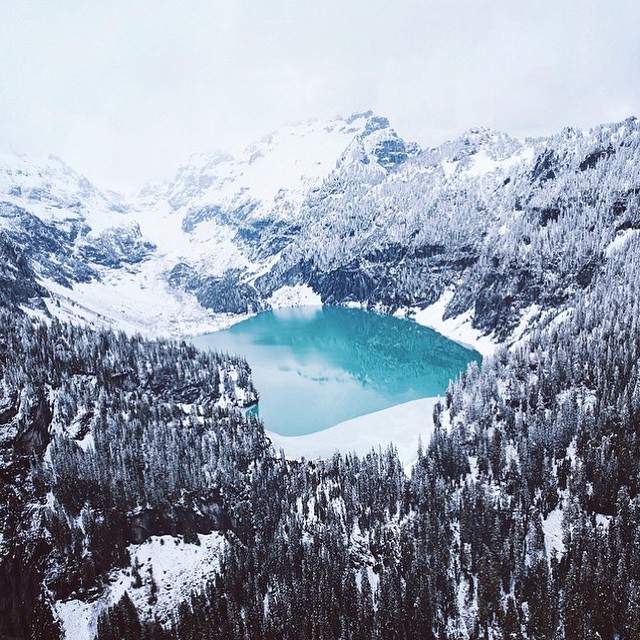
AI adoption is no longer a distant goal—it’s a pressing reality for businesses across the spectrum. As organizations chart their course through this technological revolution, a clear roadmap becomes essential. This guide explores the key milestones and considerations for navigating the AI adoption journey.
Assessing AI Readiness
The first step on any AI adoption roadmap is a thorough assessment of organizational readiness. This involves more than just evaluating technical capabilities—it requires a holistic look at the entire business ecosystem.
Data infrastructure forms the foundation of AI readiness. Companies must ensure they have robust systems for collecting, storing, and managing high-quality data. Without this groundwork, even the most sophisticated AI tools will falter.
Equally important is the human element. Does the organization have the right skills and talent to implement and manage AI systems? This often requires a mix of technical expertise and domain knowledge. Many companies find they need to invest in training programs or bring in specialized talent to bridge the skills gap.
Cultural readiness is another critical factor. Is there buy-in from leadership and employees? Resistance to change can derail AI initiatives before they even begin. Building a culture that embraces innovation and is open to AI-driven transformation is crucial for success.
Identifying High-Impact Use Cases
With a solid foundation in place, the next step is identifying the right AI use cases. The keyword here is “impact.” Organizations should focus on areas where AI can deliver tangible business value.
This process often starts with pain points. Where are the bottlenecks in current operations? Which tasks are time-consuming or error-prone? These areas often present prime opportunities for AI-driven optimization.
Another approach is to look for ways AI can enhance existing products or services. This might involve adding personalization features, improving predictive capabilities, or streamlining customer interactions.
It’s important to strike a balance between ambition and practicality. While moonshot projects can be exciting, starting with more modest, achievable goals often leads to quicker wins and builds momentum for larger initiatives.
Building the AI Infrastructure
With use cases identified, the focus shifts to building the necessary infrastructure. This goes beyond just selecting AI tools—it involves creating an ecosystem that can support ongoing AI development and deployment.
Cloud platforms often play a central role in this infrastructure. They offer the scalability and flexibility needed to handle the computational demands of AI workloads. Many organizations opt for a hybrid approach, combining on-premises systems with cloud resources.
Data pipelines are another critical component. These systems ensure a steady flow of high-quality, relevant data to feed AI models. Robust data governance practices are essential to maintain data integrity and comply with regulatory requirements.
Piloting and Scaling
The AI adoption journey is an iterative process. Starting with pilot projects allows organizations to test their assumptions, refine their approach, and demonstrate value before committing to full-scale deployment.
Successful pilots pave the way for scaling AI initiatives across the organization. This scaling process often reveals new challenges, from integrating AI systems with legacy infrastructure to managing the increased complexity of multiple AI projects.
As AI becomes more deeply embedded in business operations, new governance structures may be needed. This might include establishing AI ethics committees, developing guidelines for responsible AI use, and implementing monitoring systems to ensure AI systems are performing as intended.
Fostering Continuous Learning
The AI world is evolving rapidly. To stay ahead, organizations need to foster a culture of continuous learning and adaptation. This involves staying abreast of emerging AI technologies, regularly reassessing AI strategies, and being willing to pivot when necessary.
Collaboration plays a key role in this learning process. Partnerships with academic institutions, tech companies, or industry consortia can provide valuable insights and access to cutting-edge AI capabilities.
The AI adoption roadmap is not a linear journey—it’s a dynamic process that requires ongoing commitment and adaptation. By focusing on readiness, identifying impactful use cases, building robust infrastructure, and fostering a culture of learning, organizations can navigate the complexities of AI adoption and unlock its transformative potential.