The Power of Predictive Analytics: Driving Business Decisions with AI
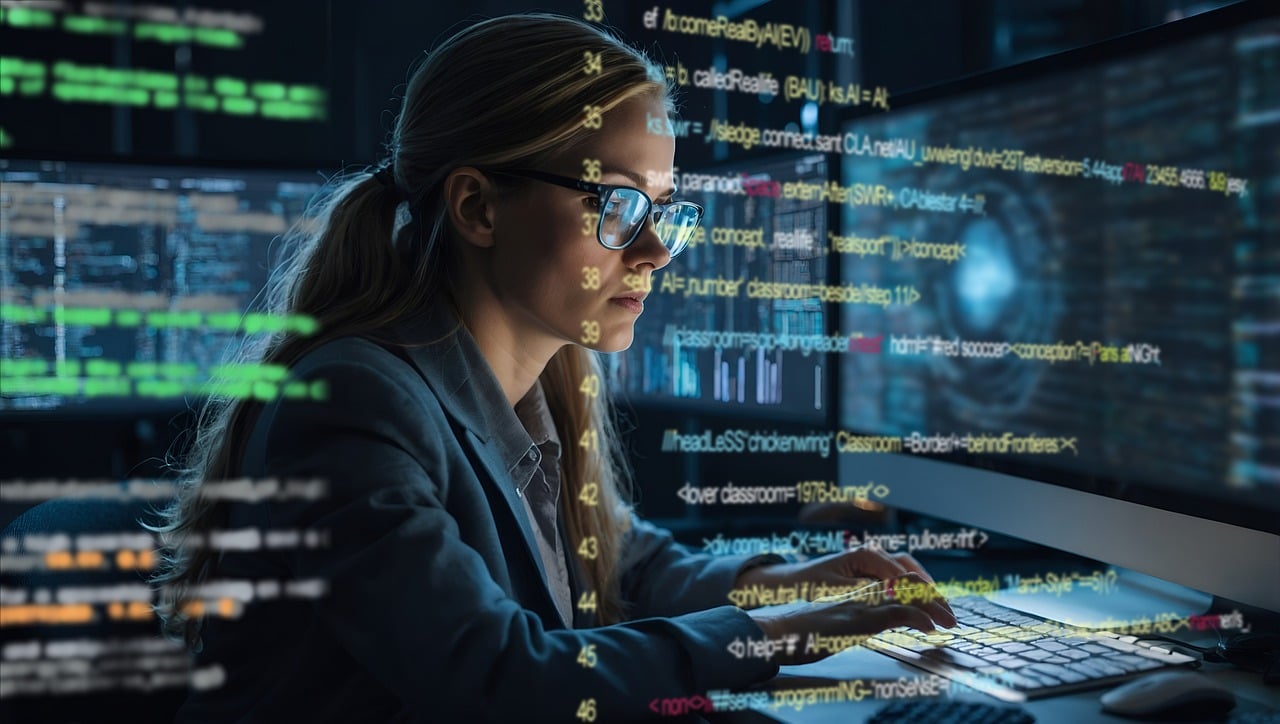
Imagine having a tool that could reveal future market trends, customer preferences, and potential risks. For modern businesses, predictive analytics powered by AI offers that capability. This advanced technology is changing how companies operate, turning raw data into actionable insights.
Gone are the days when business decisions relied solely on gut feelings and backward-looking reports. Today, predictive models analyze vast amounts of data, uncovering patterns that are invisible to the human eye. From retail giants optimizing inventory to financial institutions detecting fraud before it happens, predictive analytics is becoming an essential tool across industries.
But how exactly is this technology changing business decision-making? And what challenges do companies face in using its potential? Let’s explore the world of predictive analytics and examine its real-world applications.
Understanding Predictive Analytics
Predictive analytics uses statistical algorithms and machine learning techniques to identify the likelihood of future outcomes based on historical data. When combined with AI, these tools can process vast amounts of data, recognize patterns, and generate insights that would be impossible for humans to discern manually.
Key components of predictive analytics:
- Data collection and preparation
- Statistical modeling
- Machine learning algorithms
- Data visualization
Enhancing Customer Relationship Management
One of the most impactful applications of predictive analytics is in Customer Relationship Management (CRM). By analyzing customer data, businesses can predict future behavior and tailor their strategies accordingly.
Applications:
- Customer churn prediction
- Personalized product recommendations
- Lifetime value forecasting
Companies using predictive analytics in CRM report up to 30% higher conversion rates and 50% lower customer acquisition costs.
Optimizing Supply Chain Management
Predictive analytics can significantly improve supply chain efficiency by forecasting demand, optimizing inventory levels, and predicting potential disruptions.
Key benefits:
- Reduced stockouts and overstocking
- Improved demand forecasting accuracy
- Proactive risk management
Enhancing Financial Decision Making
In the financial sector, predictive analytics is used for everything from credit scoring to fraud detection. AI-powered models can analyze vast amounts of financial data to identify trends and anomalies.
Applications:
- Credit risk assessment
- Fraud detection and prevention
- Investment portfolio optimization
Improving Healthcare Outcomes
Predictive analytics is transforming healthcare by enabling early disease detection, personalized treatment plans, and more efficient resource allocation.
Key areas of impact:
- Disease risk prediction
- Treatment outcome forecasting
- Hospital readmission prevention
Studies show that predictive models can identify high-risk patients with up to 30% more accuracy than traditional methods.
Building New Marketing Strategies
AI-powered predictive analytics is helping marketers target the right customers with the right message at the right time, significantly improving campaign effectiveness.
Applications:
- Customer segmentation
- Campaign performance prediction
- Content optimization
Marketers using predictive analytics report up to 40% higher ROI on their campaigns.
Enhancing Human Resources Management
Predictive analytics is also making waves in HR, helping companies make data-driven decisions about recruitment, retention, and employee development.
Key applications:
- Candidate success prediction
- Employee churn forecasting
- Workforce planning
Companies using predictive analytics in HR report up to 25% reduction in employee turnover.
Challenges in Implementing Predictive Analytics
While the benefits are clear, implementing predictive analytics comes with its own set of challenges:
- Data quality and availability: Predictive models are only as good as the data they’re trained on.
- Skills gap: There’s a shortage of professionals with the necessary data science and AI skills.
- Integration with existing systems: Implementing predictive analytics often requires significant changes to existing IT infrastructure.
- Ethical considerations: The use of AI in decision-making raises important questions about fairness and transparency.
Best Practices for Using Predictive Analytics
To maximize the value of predictive analytics in your organization:
- Start with clear business objectives: Identify specific problems you want to solve with predictive analytics.
- Ensure data quality: Invest in data cleaning and preparation processes.
- Choose the right tools: Select analytics platforms that align with your technical capabilities and business needs.
- Build cross-functional teams: Combine data science expertise with domain knowledge.
- Focus on interpretability: Ensure your models provide explainable results to build trust and enable action.
- Continuously monitor and refine: Regularly assess model performance and update as needed.
- Address ethical concerns: Implement guidelines to ensure fair and responsible use of predictive analytics.
Predictive analytics, powered by AI, is changing how businesses make decisions. From customer relations to supply chain management, finance to healthcare, its applications are vast and impactful. As AI technology continues to advance, the power and accuracy of predictive analytics will only grow, making it an essential tool for businesses looking to stay competitive in the data-driven future.
However, it’s crucial to remember that predictive analytics is a tool to augment human decision-making, not replace it entirely. The most successful implementations of predictive analytics combine the power of AI with human expertise and intuition, leading to more informed, data-driven decision-making across the organization.