Unlocking the Future: 7 Strategies for Effectively Managing AI Risks
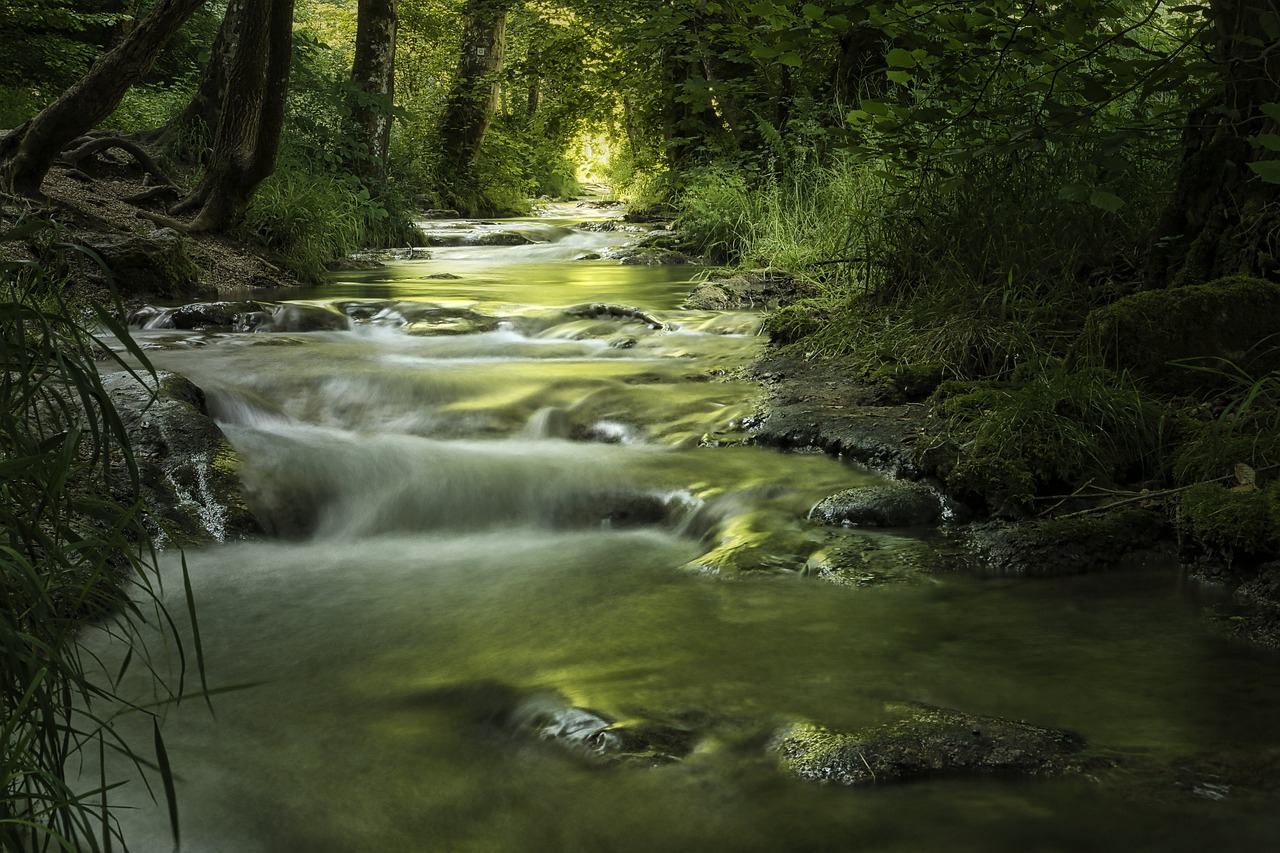
Artificial Intelligence (AI) is reshaping industries, economies, and societies at an unprecedented pace. From autonomous vehicles and healthcare diagnostics to financial algorithms and smart cities, AI is pushing the boundaries of what technology can achieve. However, as the integration of AI accelerates, so do the risks associated with its use. Misaligned algorithms, unintended biases, privacy violations, and even AI autonomy pose significant concerns. To harness AI’s potential while mitigating these risks, we must explore strategic approaches to effectively manage the potential dangers it presents. This article outlines seven key strategies for navigating the future of AI safely and responsibly.
The Rise of AI and Its Associated Risks
AI has the power to transform industries, solve complex problems, and improve daily life in ways we couldn’t have imagined just a decade ago. Yet, it comes with risks that could have profound societal impacts. As AI systems become increasingly autonomous and complex, managing these risks has never been more urgent.
The concerns surrounding AI range from technical issues—such as algorithmic biases and system errors—to ethical and regulatory challenges. If left unchecked, these risks could lead to significant disruptions, including breaches of privacy, discrimination, or even misuse in security systems.
To effectively unlock the future of AI, it’s critical to strike a balance between innovation and risk management. Below, we explore seven strategies to manage these risks while ensuring AI continues to serve humanity’s best interests.
Understanding AI Risks: What Are We Protecting Against?
Before diving into risk management strategies, it’s essential to understand the broad categories of risks AI systems pose. They can be grouped into several main areas:
- Ethical Risks: AI systems may perpetuate or exacerbate biases, making decisions that reflect societal inequalities. AI may also raise questions about privacy, surveillance, and the loss of human autonomy.
- Technical Risks: AI systems, particularly deep learning models, can malfunction, produce errors, or be vulnerable to adversarial attacks. A key concern is the “black box” nature of many AI algorithms, making their decision-making processes opaque.
- Regulatory Risks: AI’s rapid pace of advancement often outstrips existing regulatory frameworks. A lack of standards for AI development and deployment can lead to inconsistent safety and ethical practices across industries.
- Economic Risks: AI may disrupt job markets by automating tasks traditionally performed by humans, leading to economic inequality and displacement of workers.
Understanding these risks is the first step in mitigating them. Now, let’s dive into the strategies for managing these challenges effectively.
1. Promoting Transparency and Explainability
One of the most significant challenges with AI systems is the “black box” problem—where AI makes decisions based on complex computations that even its creators may not fully understand. This opacity creates ethical and technical concerns, particularly when AI is used in sensitive areas like healthcare, law enforcement, and finance.
To manage these risks, we need to promote transparency and explainability in AI systems. Developers should build AI models that allow stakeholders to understand how decisions are made, ensuring accountability and trust. By enhancing the transparency of algorithms, we can foster greater public confidence and mitigate the risks of unintended harm.
How to Implement This:
- Prioritize the development of explainable AI (XAI) models, which can provide human-understandable insights into how decisions are made.
- Require organizations to disclose the data sources and decision-making processes of AI systems, particularly in high-stakes industries like healthcare and finance.
- Establish ethical guidelines for transparency in AI design and deployment, ensuring that users and regulators can audit AI decisions.
2. Addressing Bias in AI Systems
Bias is one of the most well-documented risks of AI systems. Since AI learns from data, any biases present in the data can influence the algorithm’s decisions. These biases can lead to discriminatory outcomes, particularly in areas like hiring, law enforcement, and financial services.
Managing bias requires a multi-faceted approach. It begins with identifying and correcting biases in training datasets but extends to ongoing monitoring and adjustments as AI systems are deployed in the real world. The key is to ensure that AI does not perpetuate or amplify existing inequalities.
How to Implement This:
- Conduct bias audits during the development and deployment of AI systems, with a focus on identifying discriminatory patterns in training data.
- Develop diverse and representative datasets to ensure that AI systems are trained on data that reflects different demographic groups.
- Implement continuous monitoring mechanisms that track AI decision-making in real time, allowing for bias detection and correction after deployment.
3. Enhancing Human Oversight and Collaboration
AI systems, particularly those that make autonomous decisions, can reduce human control over critical processes. However, effective risk management requires maintaining human oversight, especially in scenarios where AI decisions have significant real-world consequences.
A balanced approach involves using AI to augment human decision-making, not replace it. By enhancing human-AI collaboration, we can ensure that humans remain in control, using AI to assist rather than dictate outcomes.
How to Implement This:
- Develop AI systems that include human-in-the-loop (HITL) frameworks, where AI decisions are reviewed and validated by humans before being implemented.
- Train AI users and operators to effectively interpret and act on AI recommendations, ensuring that human oversight is not just a formality but an integral part of the decision-making process.
- Design AI systems with built-in safeguards that allow humans to override AI decisions when necessary.
4. Building Ethical AI Governance Frameworks
Governance is essential for managing the risks posed by AI. However, the current regulatory landscape often lags behind AI advancements, creating a patchwork of rules that vary by region and industry. A comprehensive and cohesive governance framework can ensure that AI systems are developed, deployed, and monitored in line with ethical standards.
Governance frameworks should encompass ethical guidelines, technical standards, and legal regulations that align with the societal values we aim to uphold. Importantly, these frameworks should be flexible enough to adapt to the evolving nature of AI technologies.
How to Implement This:
- Establish industry-specific ethical standards that guide the development and use of AI systems, focusing on areas such as data privacy, fairness, and accountability.
- Encourage the creation of national and international AI regulatory bodies that work together to develop consistent global standards for AI governance.
- Support the formation of AI ethics boards within organizations to review and guide the ethical use of AI in business practices.
5. Ensuring Data Privacy and Security
AI systems rely on vast amounts of data to function, making them a significant risk to privacy. Without strong data protection measures, personal information could be misused or exposed to malicious attacks. Additionally, AI systems themselves can become targets for cyberattacks, potentially leading to breaches of critical systems.
To manage these risks, organizations must prioritize robust data privacy and cybersecurity practices. This includes adhering to data protection regulations, encrypting sensitive information, and safeguarding AI systems against hacking and manipulation.
How to Implement This:
- Adopt privacy-by-design principles when developing AI systems, ensuring that data protection is integrated into the design phase rather than being an afterthought.
- Encrypt sensitive data used by AI systems and apply anonymization techniques to minimize the risk of data breaches.
- Implement multi-layered cybersecurity strategies that protect AI systems from external threats, including adversarial attacks and data tampering.
6. Developing AI Auditing and Monitoring Mechanisms
AI systems, particularly those that operate in dynamic environments, need continuous monitoring to ensure they are functioning as intended. Auditing AI systems—both before and after deployment—can help identify risks such as algorithmic bias, system errors, or changes in performance.
AI audits should involve third-party assessments to provide an objective view of the system’s behavior. In addition, regular monitoring ensures that AI systems remain aligned with their intended goals, even as external conditions change.
How to Implement This:
- Develop a framework for AI audits that evaluates the system’s ethical, technical, and performance criteria before it is deployed.
- Implement real-time monitoring tools that track AI decisions and outcomes, ensuring that any issues are detected and addressed promptly.
- Encourage independent, third-party audits to ensure unbiased evaluations of AI systems, especially in critical sectors like healthcare and finance.
7. Preparing for AI’s Socioeconomic Impact
Beyond the immediate technical and ethical risks, AI has broader socioeconomic implications, particularly concerning employment and income inequality. As AI systems automate tasks across various industries, they have the potential to displace workers and reshape job markets.
Managing these risks requires proactive planning for AI’s societal impact. This could involve re-skilling programs, social safety nets, and policy interventions to ensure that the benefits of AI are shared equitably across society.
How to Implement This:
- Develop workforce retraining programs that equip workers with the skills needed for jobs in an AI-driven economy, focusing on areas where human intelligence complements AI.
- Encourage policies that promote job creation in emerging industries powered by AI, ensuring that economic opportunities are distributed widely.
- Establish social safety nets that support workers displaced by AI, providing them with financial assistance and access to retraining opportunities.
The Future of AI with Responsible Risk Management
Artificial Intelligence offers transformative potential, but with great power comes great responsibility. The future of AI is not
predetermined; it will be shaped by the decisions we make today regarding how to manage its risks. By adopting the strategies outlined in this article—promoting transparency, addressing bias, enhancing human oversight, building governance frameworks, ensuring privacy, developing auditing mechanisms, and preparing for socioeconomic impacts—we can unlock AI’s potential while safeguarding society from its unintended consequences.
As we move forward, it’s essential to foster collaboration between technologists, policymakers, businesses, and civil society. Only by working together can we ensure that AI’s future is one that benefits all of humanity.