Who’s Accountable? Addressing Liability Gaps in AI Systems
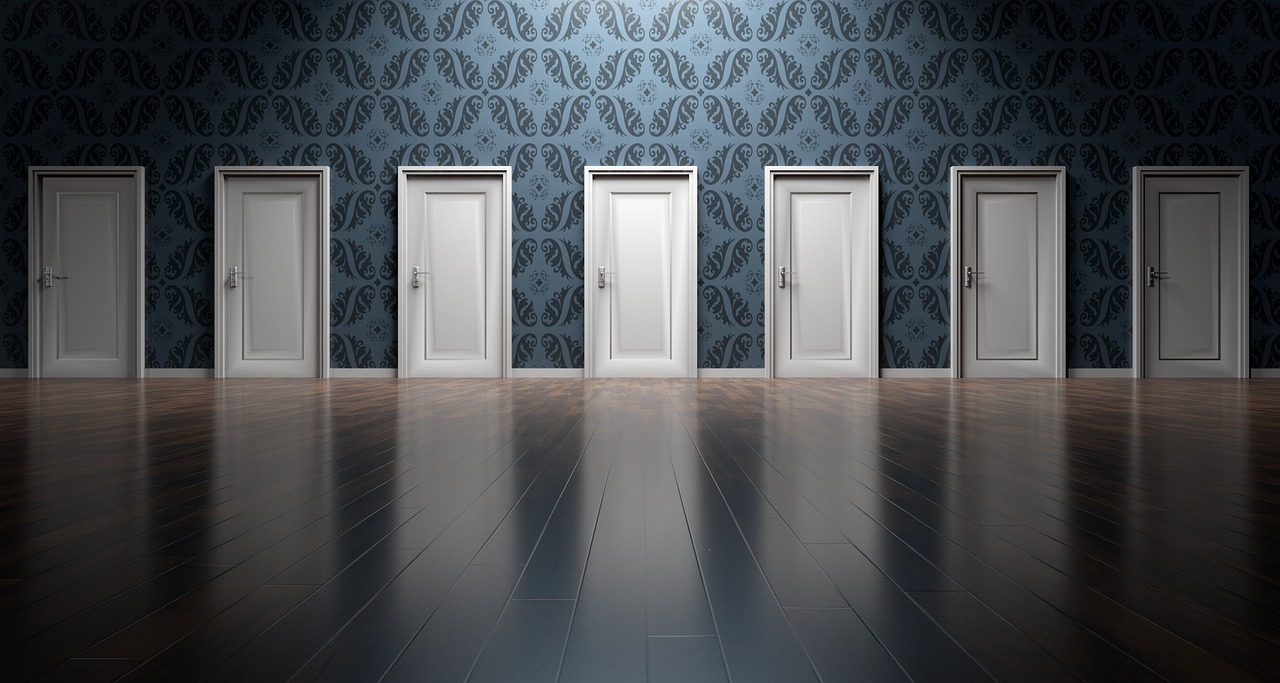
As artificial intelligence (AI) continues to evolve and become integrated into various industries—from healthcare and finance to autonomous vehicles and consumer technology—it brings with it unprecedented benefits and risks. AI systems have the potential to revolutionize how we live and work, but they also raise significant questions about accountability and liability when things go wrong. In the case of accidents, errors, or harmful decisions made by AI, determining who is responsible can be complex. The challenge of assigning liability in AI-driven systems is critical to ensuring trust, fairness, and safety in an increasingly automated world.
This article explores the key issues surrounding accountability and liability in AI systems, addressing the challenges posed by liability gaps and discussing potential solutions for ensuring responsible AI deployment.
The Complexity of Accountability in AI
Artificial intelligence, particularly machine learning systems, can operate with a high degree of autonomy. They make decisions based on data, patterns, and algorithms that may not always be fully understandable or predictable, even to their developers. This autonomy blurs traditional lines of accountability because, unlike a human decision-maker, an AI system doesn’t have moral or legal responsibility.
In conventional systems, when a product or service malfunctions, accountability typically falls to a specific individual or entity, such as the manufacturer or service provider. But in AI-driven systems, several parties could potentially share liability, including:
- The developers who designed the algorithm
- The company that deployed the AI system
- The data providers who supplied the training data
- The end-user who operates or interacts with the AI
This fragmentation creates a liability gap, raising the question: Who is accountable when AI systems fail?
Key Areas of Concern in AI Accountability
1. Autonomous Systems and Unpredictable Behavior
AI systems, particularly those based on machine learning, are designed to learn and evolve from the data they are exposed to. While this adaptability allows them to perform tasks more efficiently, it also means that their actions can become unpredictable over time. For example, an autonomous vehicle might make a decision in a real-world scenario that was not explicitly programmed by its developers. If such a decision leads to an accident, the system’s unpredictability complicates the process of assigning liability.
In such cases, is the AI developer responsible for an unforeseen decision made by the system? Or does liability fall on the vehicle’s owner or the company that deployed the AI? Without clear lines of accountability, victims of AI-related harm may struggle to seek justice or compensation.
2. The Black Box Problem
Many AI systems operate as “black boxes,” where their decision-making processes are not fully transparent or understandable, even to those who created them. Neural networks, for example, can make highly accurate predictions or decisions, but their internal workings are often opaque. This lack of transparency raises concerns about explainability, particularly when AI systems are involved in critical sectors such as healthcare or criminal justice.
If an AI system makes an incorrect diagnosis or an unfair decision, how can we determine whether it was a flaw in the algorithm, biased training data, or improper deployment? The black box nature of AI complicates efforts to pinpoint the source of an error, making it difficult to assign liability.
3. Bias and Discrimination in AI Systems
Bias in AI systems is a well-documented issue, particularly in systems that rely on large datasets for training. AI algorithms can unintentionally perpetuate existing biases in the data, leading to discriminatory outcomes. For example, AI systems used in hiring processes have been found to favor certain demographic groups over others, and facial recognition technology has shown higher error rates for people of color.
When bias leads to harm or discrimination, determining accountability becomes challenging. Who is responsible for biased outcomes—the developers who built the system, the data providers, or the organizations that deployed it without properly testing for fairness? Addressing these questions is critical to ensuring that AI systems are used responsibly and ethically.
4. AI and Human Oversight
Many AI systems are deployed with some level of human oversight, where humans have the ability to intervene or override the AI’s decisions. However, the degree of human control varies significantly between applications. In some cases, human operators may rely too heavily on AI, assuming that the system’s decision is correct without fully understanding its limitations. This phenomenon, known as “automation bias,” can lead to errors and accidents if the human operator fails to intervene when necessary.
In these scenarios, should the blame fall on the human operator for not exercising adequate judgment, or does the liability lie with the developers and designers of the AI system? The shared nature of decision-making between humans and AI creates further ambiguity in assigning responsibility.
Legal and Regulatory Challenges
1. Current Legal Frameworks
Existing legal frameworks were developed long before the rise of autonomous AI systems, and they often fall short when applied to AI-related incidents. Most legal systems are based on the assumption that responsibility lies with a human agent—either an individual or a corporation. However, AI systems introduce new complexities that do not fit neatly into traditional liability models.
In many jurisdictions, the law holds manufacturers strictly liable for harm caused by defective products. However, in the case of AI, the “product” is not static—it evolves over time as it learns from data. This raises the question of whether traditional product liability laws can be applied to AI systems that continuously change their behavior.
2. The Need for New Legislation
To address these gaps, there is growing recognition of the need for updated legal frameworks specifically designed for AI. Some governments and regulatory bodies are beginning to explore potential solutions. For example, the European Union has proposed an AI regulatory framework that includes provisions for high-risk AI systems and outlines specific requirements for transparency, accountability, and human oversight.
Potential approaches to regulating AI liability include:
- Strict Liability for certain types of AI systems, particularly those that are autonomous or involved in high-risk activities, such as self-driving cars or AI in healthcare.
- Mandatory Insurance for AI developers and companies deploying AI systems, ensuring that victims can receive compensation in the event of harm.
- AI Certification Programs that require developers to meet certain safety, ethical, and transparency standards before deploying AI systems in the market.
However, these regulatory efforts are still in their early stages, and much work remains to be done to establish clear and consistent guidelines across jurisdictions.
Proposed Solutions to Address Liability Gaps
1. Clear Chain of Accountability
One of the most effective ways to address liability gaps is to establish a clear chain of accountability for AI systems. This could involve defining the roles and responsibilities of all parties involved in the design, deployment, and operation of AI. Developers, data providers, and end-users should all have clearly defined obligations to ensure that AI systems are safe, ethical, and transparent.
2. Improved Transparency and Explainability
To mitigate the challenges posed by the black box problem, AI systems should be designed with transparency and explainability in mind. Developers can use techniques such as explainable AI (XAI) to ensure that AI decisions are understandable to both technical and non-technical users. This would allow stakeholders to identify the source of an error or bias more easily, facilitating clearer accountability.
3. Human-AI Collaboration Frameworks
AI systems should be designed to complement human decision-making, with clear guidelines on when and how humans should intervene. Creating robust human-AI collaboration frameworks would reduce the risks associated with automation bias and ensure that human operators retain ultimate control over critical decisions.
4. Ethical AI Guidelines and Standards
Developing and adopting industry-wide ethical AI guidelines can help prevent harmful or biased outcomes. These standards should cover everything from the collection and use of data to the design and deployment of AI systems. By embedding ethics into the AI development process, companies can reduce the likelihood of liability issues arising from discriminatory or unsafe AI behavior.
Building a Framework for Accountability
As AI systems become more integrated into society, addressing the liability gaps in AI accountability is essential for fostering public trust and ensuring responsible innovation. The complexity of AI systems makes it challenging to assign responsibility when things go wrong, but by improving transparency, establishing clear legal frameworks, and fostering collaboration between humans and AI, we can create a more accountable future.
Ultimately, building accountability into AI systems is not just a legal or regulatory challenge—it is a moral imperative. As AI continues to shape our world, ensuring that its development and deployment are aligned with ethical principles and clear lines of responsibility is essential for realizing the full potential of AI while safeguarding the rights and safety of individuals.